27 February 2024: Clinical Research
Predictors of Mortality in Veterans with Amyotrophic Lateral Sclerosis: Respiratory Status and Speech Disorder at Presentation
Meheroz H. Rabadi1ABEF*, Kimberly C. Russell1B, Chao Xu2CDDOI: 10.12659/MSM.943288
Med Sci Monit 2024; 30:e943288
Abstract
BACKGROUND: There is a lack of accurate models to predict amyotrophic lateral sclerosis (ALS) disease course and outcomes. As a result, risk assessment and counseling, the timing of interventions, and their stratification in clinical trials are difficult. This study aimed to evaluate the association between symptoms at presentation and mortality.
MATERIAL AND METHODS: A single veterans hospital reviewed the electronic records of 105 veterans with ALS who were periodically followed in our ALS clinic between 2010 and 2021. A survival decision tree (≤3 or >3 years) was generated based on the statistical median survival of our data. The variables known to influence survival when alive were compared to patients who died.
RESULTS: The (mean±SD) age at onset was 62±11 years, M/F ratio 101: 4, and 90% were non-Hispanic whites. The initial score for the Amyotrophic Lateral Sclerosis Functional Rating Scale-Revised (ALSFRS-R) was 31±8.3. Dysarthria and shortness of breath (SOB) were present on initial presentation in 52 (49.5%) and 32 (30.5%) patients, respectively. Deaths occurred in 80 (76.2%) patients during the study period. The main cause of death was respiratory disease (failure and pneumonia, n=43 53.75%). Patients survived for >3 years on initial presentation with normal respiration and speech, compared to ≤3 years of survival in patients with dysarthria and SOB, irrespective of age.
CONCLUSIONS: This study suggests that for veterans with ALS, the main predictors of shorter survival were respiratory status and speech disorder on initial presentation to the clinic.
Keywords: Veterans, Amyotrophic Lateral Sclerosis, Survival, Clinical Decision Rules
Background
Amyotrophic lateral sclerosis (ALS) is a rare and progressively fatal neurodegenerative disease with a worldwide incidence of 1.6 cases per 100 000 patients [1]. It is caused by loss of motor neurons in the brain, brainstem, and spinal cord [1], which leads to painless voluntary muscle weakness presenting as lower motor neuron findings from muscle atrophy/wasting (amyotrophy) and fasciculations, as well as upper motor neuron findings of hyperreflexia and spasticity from axonal loss involving the corticospinal tracts in the lateral spinal cord columns (lateral sclerosis) [1–3]. ALS can occur at any adult age, with 10–15% of patients having a family history of ALS [2], and approximately 15% of patients developing frontotemporal dementia (FTD) [4]. The risk of FTD is greater in patients with bulbar onset of the disease [5]. ALS is a clinical diagnosis based on the revised El Escorial criteria [6], which require the presence of: (i) clinical, electrophysical, or neuropathologic evidence of lower motor neuron degeneration, and (ii) clinical, electrophysical, or neuropathologic evidence of upper motor neuron degeneration, and evidence of progressive spread of symptoms and signs within a region or to other regions. This is present in the absence of electrophysical, neuropathological, or radiological evidence of other disease processes that can explain the patient’s motor degeneration. Electrophysiological findings include fibrillation and positive sharp waves (suggesting acute denervation), long-duration complex motor unit action potential (cMUAP, suggesting chronic denervation), and large amplitude cMUAP (suggesting re-innervation) in the presence of normal sensory action potentials [7]. Patients usually die from respiratory failure within 2–4 years from the initial diagnosis of involvement of respiratory muscles [1,2].
Treatment with Riluzole, Edaravone, and, recently, Relyvrio has a modest effect (measured in months) on survival. Symptomatic management is the main treatment in patients with ALS, as recommended by the multidisciplinary ALS team [8].
Since ALS is a heterogeneous disease with limb-onset, bulbar onset, and frontotemporal dementia, the survival of patients with ALS varies greatly, from months to years. ALS survival at the group level has been extensively studied [9], and the patient characteristics associated with shorter survival include older age at symptoms onset, the presence of frontotemporal dementia, or a repeat expansion in the
Therefore, this retrospective study from a single veterans’ hospital of patients with ALS with out-patient follow-up between 2010 and 2021 aimed to evaluate the association between symptoms at presentation and mortality. The results may help guide: (i) prescribing appropriate treatment, (ii) instituting ventilatory and nutritional support in the early disease stage, and (iii) ordering the appropriate level of care, amount (of hours), and types of services to be set up, including in the home. This study may help form the basis for the provision of veterans’ medical care, as they make up 7% (22 million) of the US population.
Material and Methods
PARTICIPANTS:
This study was approved by the local Human Research Ethics Committee (IRB # 10358) and was exempt from veteran informed consent because of the retrospective review of the electronic medical records, veterans were still being followed clinically, and most of the veterans had died.
This single-center, retrospective, longitudinal, observational study collected the electronic data of all veterans diagnosed with definite/probable ALS by the revised El Escorial criteria [6] and supported by neurophysiology testing [7]. The veterans were periodically followed every 4, 8, and 12 months (annual) in our ALS program at the Oklahoma City VA Medical Center (OKC VAMC) from 1/1/2010 to 12/31/2021. The OKC VAMC is an ALS Regional Center of Excellence that provides ALS-specialty care with a continuum of acute, chronic, and long-term phases of the disease following the Veteran Health Administration policies. The ALS clinic set-up involves the veteran with ALS meeting in a room with multidisciplinary team members, comprising a neurologist, respiratory physician, physician assistant, occupational, speech and respiratory therapists, dietician, prosthetic department, and social worker, who is also the clinic coordinator.
ELIGIBILITY CRITERIA:
The inclusion criteria were complete electronic medical records of veterans regularly followed in our ALS clinic, including the causes and time (years) of death. The exclusion criterion was incomplete electronic medical records, including a lack of documentation of both causes and the time (year) of death.
STUDY DESIGN: SINGLE-CENTER:
The demographic and clinical variables collected were age at diagnosis, sex, race/ethnicity, body mass index (BMI) [17], ALS status (age at ALS onset, clinical ALS subtypes [appendicular, bulbar, and respiratory]), and duration of the disease. Veterans were considered dysarthric if they had slurred speech and on clinical evaluation were found to have buccal, lingual, and/or pharyngeal weakness, wasting, and fasciculation. Furthermore, a speech pathologist confirmed dysarthria by assessing articulation, phonation, prosody, resonance, and respiratory airflow. Veterans were considered to have respiratory insufficiency or shortness of breath if they had shortness of breath at rest (orthopnea) or on exertion, or needed 2 or more pillows to sleep, which limited their ability to undertake usual activities of daily living. On clinical evaluation, they were found to have tachypnea and/or tachycardia with accessory muscle use and the presence of paradoxical breathing. Supine FVC helped confirm the impression of respiratory insufficiency (diaphragmatic weakness). In addition, we recorded the presence of co-morbidities known to cause disability and death in the general US population, such as hypertension, hyperlipidemia, diabetes mellitus, and current smoking [18], and ALS-related complications such as depression [17].
Initial and follow-up evaluations included the Mini-Mental State Examination (MMSE) for cognition [19], which is a widely used, reliable, and valid scale [20], with scores ranging from 0 to 30 (a score of 26 points or greater being normal, 21–25=mild, 16–20=moderate, and 15 or less=severe cognitive impairment). The severity and progression of ALS general were measured using the ALS Functional Rating Scale-Revised (ALSFRS-R), which includes the ALS Functional Rating Scale 10 questions plus 2 additional questions related to the respiratory state. The score per question ranges from 0 to 4, resulting in a maximum possible score of 48 on the ALSFRS-R [21,22].
The respiratory function was measured by forced vital capacity (FVC) and maximum inspiratory and expiratory pressures (MIP and MEP), which are effort-dependent, and sniff nasal inspiratory pressure (SNIP) [23].
This study meets all STROBE guidelines and the information required is reported accordingly (see the Supplementary Checklist).
ASSESSMENT OF MORTALITY:
Mortality is a suitable outcome measure of quality as it helps identify treatable factors that can improve medical care, reduce the cost of medical care (eg, need for hospitalization), and improve survival. From an organizational standpoint, it shows an area of care that needs to be improved, interventions that work best for the patients under given circumstances, and the effectiveness of treatments such as lower hospitalization rates and compliance with best practice guidelines. Deaths were ascertained for all veterans with ALS who were followed in the clinic from 1/1/2010 and died before 12/31/2021. The causes and locations (home, long-term care facility, or hospital) of death were provided by the patient’s care provider or next of kin, who informed our clinic coordinator/social worker, and from the death certificates filed with the Oklahoma State Department of Health.
STATISTICAL ANALYSIS:
Statistical significance was considered a
Results
Table 1 presents the characteristics of 105 veterans with ALS on their initial clinic presentation/visit over 11 years. The (mean±SD) age at onset and duration from onset of symptoms to diagnosis were 62±11 and 2.6±3.3 years, respectively. The number of veterans with ALS onset types at presentation was appendicular (n=55, 52%), bulbar (n=45, 43%), and respiratory (n=5, 4.7%). The study sample consisted mostly of men (n=101, 96.2%), and 95 (91%) were non-Hispanic whites with a moderate level of ALS-disability (ALSFRS-R mean score 31±8.3, and TFIM mean score 91±25). The 2-MWT score for this study sample was 200±160 feet. The mean survival time in the study population was 4.1±3.9 years.
Figure 1 shows the survival prediction analysis decision tree used in this study. This decision tree yielded an accuracy of 0.7 (95% CI 0.587, 0.797) and Matthew’s correlation coefficient (MCC) of 0.397, which shows a good positive correlation between the predicted and true outcomes. The sensitivity for identifying worse outcomes (≤3 years survival) was 0.705, while the specificity was 0.694. In the validation set, this decision tree yielded an accuracy of 0.737, an MCC of 0.42, a sensitivity of 0.769, and a specificity of 0.667. Thus, the decision consistently exhibited good prediction performance in the training and validation sets. The decision tree showed that irrespective of the age of onset, if subjects were short of breath (SOB), their survival was ≤3 years compared to >3 years for subjects without SOB or dysarthria. If subjects had no SOB but were dysarthric and the age of onset was younger than 67 years, their survival was ≤3 years compared to >3 years in those whose age of onset was ≥67 years.
Discussion
The main findings of this single-center, retrospective, observational study on the 11-year longitudinally collected data on veterans with ALS were as follow. (i) The veteran population studied was predominantly white men in their 60s, which is like the general ALS population [24], but we found a higher percentage (43%) of bulbar involvement compared to 25–30% in the general ALS population [1]. This higher percentage reflects the older age men in our sample [25]. (ii) The veteran population had a lower level of ALS-related disability and a higher death rate. (iii) The average survival time from initial symptom presentation was 4 years. (iv) Most of the subjects died at home. (v) The personalized prediction model showed that veterans were likely to live longer (>3-years) if they had normal speech (no dysarthria) and respiratory function (no underlying SOB on exertion or rest). However, veterans who had SOB or dysarthria and were younger than 67 years at initial evaluation also had a shorter lifespan (<3 years).
We compared our survival prediction model to those proposed by Westeneng [26], Schuster [15], and Kuan Li [16]. Westeneng’s [26] prediction model’s shortcomings are: (i) Data were obtained from 14 ALS centers in Europe comprising 1936 patients. This was a heterogenous population as it comprised 3 populations and 11 referral-based registries with differing referral mechanisms “referral bias”; (ii) their Royston-Parmar model was based on 8 variables (age at onset, definite ALS, progression rate on ALS-FRS, FVC, bulbar onset, FTD, and C9Orf 72), defining 5 survival groups (very short, short, intermediate, long, and very long); (iii) missing data needed imputation methods to address the inherent biases; and (iv) they had no inclusion criteria and was based on eligibility. Thus, their model was based on a heterogeneous ALS patient population with missing data that needed imputation and had limited variables to define the survival groups. Schuster [15] used a binary log ridge regression to predict 18-month survival based on combined clinical and MRI features in 60 patients with ALS and 60 healthy volunteers. They showed that patients with ALS had widespread cortical and white matter changes on the MRI compared to healthy controls, and MRI-based measures of specific brain regions enhanced their ALS survival prediction model. However, the main problems with their prediction model are that most of the facilities do not have access to: (i) 3 Tesla MRI on which this data was based; (ii) the use of machine learning to help delineate specific brain regions; and (iii) the data were based on a single time-point to predict a short-duration survival period of 18 months. The accuracy, sensitivity, and specificity of their prediction model were 66.6%, 62.5%, and 70%, respectively, which was lower than our prediction model of 0.7, 0.70, and 0.73, respectively. Kuan [16] predicted survival based on machine learning using mean absolute error margin (MAE-margin) in 216 patients with ALS from the Canadian ALS Neuroimaging Consortium (CALSNIC). They incorporated clinical features and MRI images of cortical thickness as surrogate markers for individual survival distribution. Like the prior Schuster study, at present facilities do not have access to machine learning software and MRI of a higher Tesla strength. Likewise, Querin et al [27], in their study of 49 ALS patients, employed a multivariate Cox regression model for predicting the survival of ALS patients using clinical, 3 Tesla MRI spinal cord, and diffusion tensor imaging (DTI) data. They reported that MRI showing spinal cord atrophy was more predictive than clinical variables in survival prediction (hazard ratio of 0.68 to 1). Furthermore, Van der Burgh et al [28], in their study of 135 ALS patients, applied a deep neural network to 8 clinical features, along with some imaging features from diffusion-weighted and T1-weighted MR images, to produce a model that could classify ALS patients as short, medium, or long survivors. They found that just using 8 clinical features (or just the MRI characteristics) correctly predicted survival in 69% of cases, but the combined (clinical and MRI) features obtained 84.4% classification accuracy.
A recent meta-analysis of 115 studies involving 55 169 patients with ALS found predictors of poor prognosis were the presence of neurofilament light chain in serum and cerebrospinal fluid, presence of FTD, change in the rate of ALSFRS-R score (≥1/month), FVC less than 80, executive dysfunction, and older age at onset. The predictors of good prognosis were the presence of pure lower and upper motor neuron involvement, delay in ALS diagnosis, and higher initial ALSFRS-R score. However, sex, education level, and presence of co-morbidities such as diabetes mellitus, hypertension, statin use, non-invasive ventilation (NIV), and gastrostomy did not affect the survival of patients with ALS [29]. In our study, only 27.6% of veterans underwent PEG placement despite discussing PEG usage during their initial evaluation. The main reason for this low uptake was that some of the veterans’ preferred to continue taking their nutrition by mouth, some refused it outright as personal preference, and in some PEG placement was considered a risk due to their poor respiratory state, as the respiratory and gastrointestinal clinical providers on the ALS team were concerned the patients would eventually need continuous invasive ventilation. Gordon et al, in their study of 3885 patients with ALS in France, found increasing rates of use of NIV was associated with improved survival [30]. Only 34.3% of veterans in our study underwent NIV usage despite discussing NIV use during their initial evaluation irrespective of their respiratory status. The main reason for this poor compliance was that either they could not tolerate the various masks provided or it was an inconvenience as they were unable to put on and take off the masks because they lacked upper-extremities function.
At the individual level in our model, patient characteristics associated with shorter survival such as older age at symptom onset, bulbar onset, low BMI (<25 kg/m2), presence of dementia, [30,31] and duration of diagnostic delay (equated with slower disease progression, and longer survival) was not observed [32]. The initial symptoms presentation did not influence the course of the disease in our study. When Haverkamp et al studied 1200 patients with ALS, of whom 831 had sporadic ALS (69.2%), they found that sex and bulbar onset did not affect survival in their model. [33]
There are several limitations to the present study. First, the small sample size of 105 patients may have biased the results as the power to detect associations was reduced. Second, our clinical model did not include the biochemical or genetic factors associated with ALS survival. Third, the conclusions should be interpreted with caution when generalized to the general population of patients with ALS as veterans with ALS receive a higher standard of subsidized medical care through the Veterans Health System which may not be available to the general Oklahoma population. Finally, it would have been preferable to use the ALSFRS-R sub-scores rather than the total scores we used in the model for this study.
The study strength is the completeness of data collected by the standardized ALS registry over 11 years with no loss on periodic follow-up at 4, 8, and 12 months, with the completeness of the data captured by the standardized ALS registry preventing bias inherent in observational studies from missing data. Thus, our survival prediction model is based on clinical data collected in a real-world setting reflecting real-world experience. Our survival prediction model is practical, simple, and easy to use without the need for an MRI of high Tesla strength and the use of machine learning, which at present is not accessible to most facilities. As in oncology, ALS patients like to be informed of their expected survival. Setting realistic expectations helps in reducing the use of non-beneficial treatment, focusing on enhanced goal-consistent care and family support, and reducing costs [34,35]. Finally, we look at the role of chemical exposure and ALS in veterans, as pesticides are well-recognized risk factors for neurodegenerative diseases, [36], especially Parkinson disease. The VA assumes ALS diagnosed in all veterans is related to their service, irrespective of the period of service, based on the summary report by the US National Institutes of Medicine [37]. Agent orange (dioxin) exposure was present in 20% of our ALS patients and had no significant effect on survival or influence on the prediction model.
Conclusions
This study’s prediction model suggests that veterans with ALS on initial presentation to the clinic with no concerns of SOB and dysarthria survived longer than those who had SOB, were dysarthric, and were younger than 67 years of age.
References
1. Kiernan MC, Vucic S, Cheah BC, Amyotrophic lateral sclerosis: Lancet, 2011; 377(9769); 942-55
2. van Es MA, Hardiman O, Chio A, Amyotrophic lateral sclerosis: Lancet, 2017; 390(10107); 2084-98
3. Brotman RG, Moreno-Escobar MC, Joseph J, Pawar G, Amyotrophic lateral sclerosis: StatPearls [Internet] June 20, 2023, Treasure Island (FL), StatPearls Publishing
4. Ringholz GM, Appel SH, Bradshaw M, Prevalence and patterns of cognitive impairment in sporadic ALS: Neurology, 2005; 65(4); 586-90
5. Chiò A, Moglia C, Canosa A, ALS phenotype is influenced by age, sex, and genetics: A population-based study: Neurology, 2020; 94; e802-10
6. Brooks BR, Miller RG, Swash M, Munsat TLWorld Federation of Neurology Research Group on Motor Neuron Disease, El Escorial revisited: Revised criteria for the diagnosis of amyotrophic lateral sclerosis: Amyotroph Lateral Scler Other Motor Neuron Disord, 2000; 1(5); 293-99
7. Lenglet T, Camdessanché JP, Amyotrophic lateral sclerosis or not: Keys for the diagnosis: Rev Neurol (Paris), 2017; 173(5); 280-87
8. Miller RG, Jackson CE, Kasarskis EJQuality Standards Subcommittee of the American Academy of Neurology, Practice parameter update: The care of the patient with amyotrophic lateral sclerosis: multidisciplinary care, symptom management, and cognitive/behavioral impairment (an evidence-based review): Report of the Quality Standards Subcommittee of the American Academy of Neurology: Neurology, 2009; 73(15); 1227-33
9. Chio A, Logroscino G, Hardiman O, Prognostic factors in ALS: A critical review: Amyotroph Lateral Scler, 2009; 10(5–6); 310-23
10. van Rheenen W, van Blitterswijk M, Huisman MH, Hexanucleotide repeat expansions in C9ORF72 in the spectrum of motor neuron diseases: Neurology, 2012; 79(9); 878-82
11. Collins GS, Ogundimu EO, Altman DG, Sample size considerations for the external validation of a multivariable prognostic model: A resampling study: Stat Med, 2016; 35(2); 214-26
12. Riley RD, Ensor J, Snell KI, External validation of clinical prediction models using big datasets from e-health records or IPD meta-analysis: Opportunities and challenges: BMJ, 2016; 353; i3140
13. Collins GS, Reitsma JB, Altman DG, Moons KG, Transparent reporting of a multivariable prediction model for individual prognosis or diagnosis (TRIPOD): The TRIPOD statement: BMJ, 2015; 350; g7594
14. Walhout R, Westeneng HJ, Verstraete E, Cortical thickness in ALS: Towards a marker for upper motor neuron involvement: J Neurol Neurosurg Psychiatry, 2015; 86(3); 288-94
15. Schuster C, Hardiman O, Bede P, Survival prediction in amyotrophic lateral sclerosis based on mri measures and clinical characteristics: BMC Neurol, 2017; 17(1); 73
16. Kuan L-H, Parnianpour P, Kushol R, Accurate personalized survival prediction for amyotrophic lateral sclerosis patients: Sci Rep, 2023; 13(1); 20713
17. Gallo V, Wark PA, Jenab M, Prediagnostic body fat and risk of death from amyotrophic lateral sclerosis: The EPIC cohort: Neurology, 2013; 80(9); 829-38
18. Calvo A, Canosa A, Bertuzzo D, Influence of cigarette smoking on ALS outcome: A population-based study: J Neurol Neurosurg Psychiatry, 2016; 87(11); 1229-33
19. Folstein MF, Folstein SE, McHugh PR, “Mini-mental state”. A practical method for grading the cognitive state of patients for the clinician: J Psychiatr Res, 1975; 12(3); 189-98
20. Tombaugh TN, McIntyre NJ, The mini-mental state examination: A comprehensive review: J Am Geriatr Soc, 1992; 40(9); 922-35
21. Cedarbaum JM, Stambler N, Malta E, The ALSFRS-R: A revised ALS functional rating scale that incorporates assessments of respiratory function. BDNF ALS Study Group (Phase III): J Neurol Sci, 1999; 169(1–2); 13-21
22. Cedarbaum JM, Stambler N, Performance of the Amyotrophic Lateral Sclerosis Functional Rating Scale (ALSFRS) in multicenter clinical trials: J Neurol Sci, 1997; 152(Suppl. 1); S1-9
23. Fregonezi G, Araujo PR, Macedo TL, Monitoring respiratory muscle strength assists in early diagnosis of respiratory dysfunction as opposed to the isolated use of pulmonary function evaluation in amyotrophic lateral sclerosis: Arq Neuropsiquiatr, 2013; 71(3); 146-52
24. Mehta P, Raymond J, Punjani R, Prevalence of amyotrophic lateral sclerosis in the United States using established and novel methodologies, 2017: Amyotroph Lateral Scler Frontotemporal Degener, 2023; 24(1–2); 108-16
25. Chiò A, Moglia C, Canosa A, Manera U, ALS phenotype is influenced by age, sex, and genetics: A population-based study: Neurology, 2020; 94(8); e802-e10
26. Westeneng HJ, Debray TPA, Visser AE, Prognosis for patients with amyotrophic lateral sclerosis: development and validation of a personalized prediction model: Lancet Neurol, 2018; 17(5); 423-33
27. Querin G, El Mendili MM, Lenglet T, Spinal cord multi-parametric magnetic resonance imaging for survival prediction in amyotrophic lateral sclerosis: Eur J Neurol, 2017; 24(8); 1040-46
28. van der Burgh HK, Schmidt R, Westeneng HJ, Deep learning predictions of survival based on MRI in amyotrophic lateral sclerosis: Neuroimage Clin, 2016; 13; 361-69
29. Su W-M, Cheng Y-F, Jiang Z, Predictors of survival in patients with amyotrophic lateral sclerosis: A large meta-analysis: EBioMedicine, 2021; 74; 103732
30. Gordon PH, Salachas F, Lacomblez L, Predicting survival of patients with amyotrophic lateral sclerosis at presentation: A 15-year experience: Neurodegener Dis, 2013; 12(2); 81-90
31. Moura MC, Novaes MR, Eduardo EJ, Prognostic factors in amyotrophic lateral sclerosis: A population-based study: PLoS One, 2015; 10(10); e0141500
32. Kjældgaard AL, Pilely K, Olsen KS, Prediction of survival in amyotrophic lateral sclerosis: A nationwide, Danish cohort study: BMC Neurol, 2021; 21(1); 164
33. Haverkamp LJ, Appel V, Appel SH, Natural history of amyotrophic lateral sclerosis in a database population. Validation of a scoring system and a model for survival prediction: Brain, 1995; 118(Pt 3); 707-19
34. Innes S, Payne S, Advanced cancer patients’ prognostic information preferences: A review: Palliat Med, 2009; 23(1); 29-39
35. Bernacki RE, Block SDAmerican College of Physicians High Value Care Task Force, Communication about serious illness care goals: A review and synthesis of best practices: JAMA Intern Med, 2014; 174; 1994-2003
36. Tanner CM, Goldman SM, Ross GW, Grate JSJ, The disease intersection of susceptibility and exposure: Chemical exposures and neurodegenerative disease risk: Alzheimers Dement, 2014; 10(3Suppl.); S213-25
37. Institute of Medicine (US), Committee on Gulf War and Health Gulf War and Health: Update of Health Effects of Serving in the Gulf War, 2010, Washington, DC, National Academies Press
Tables
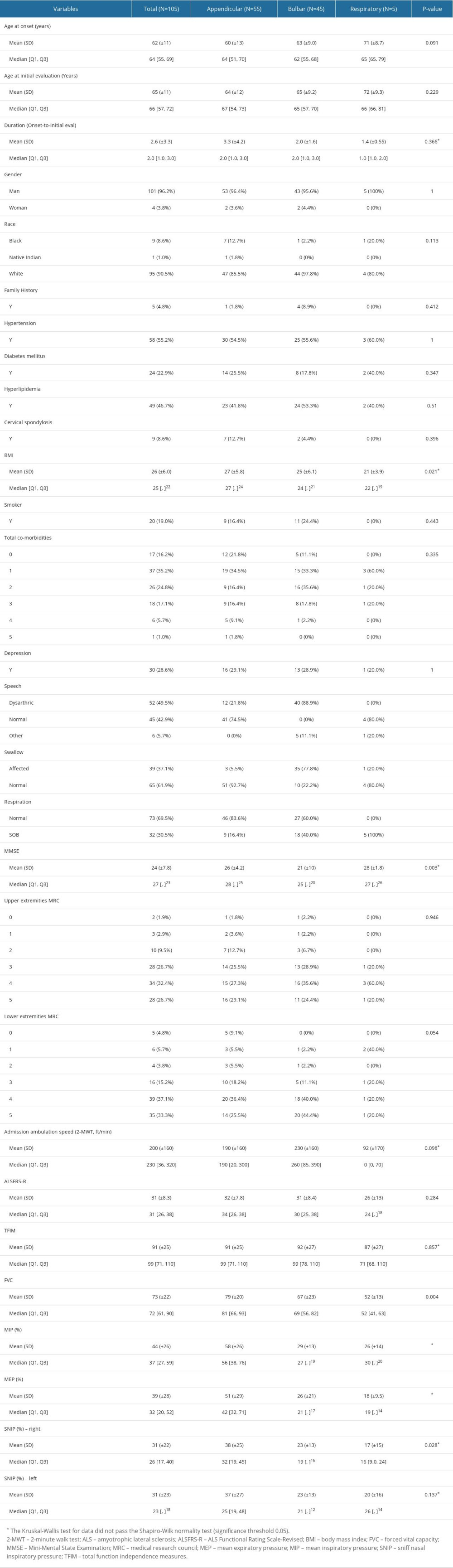
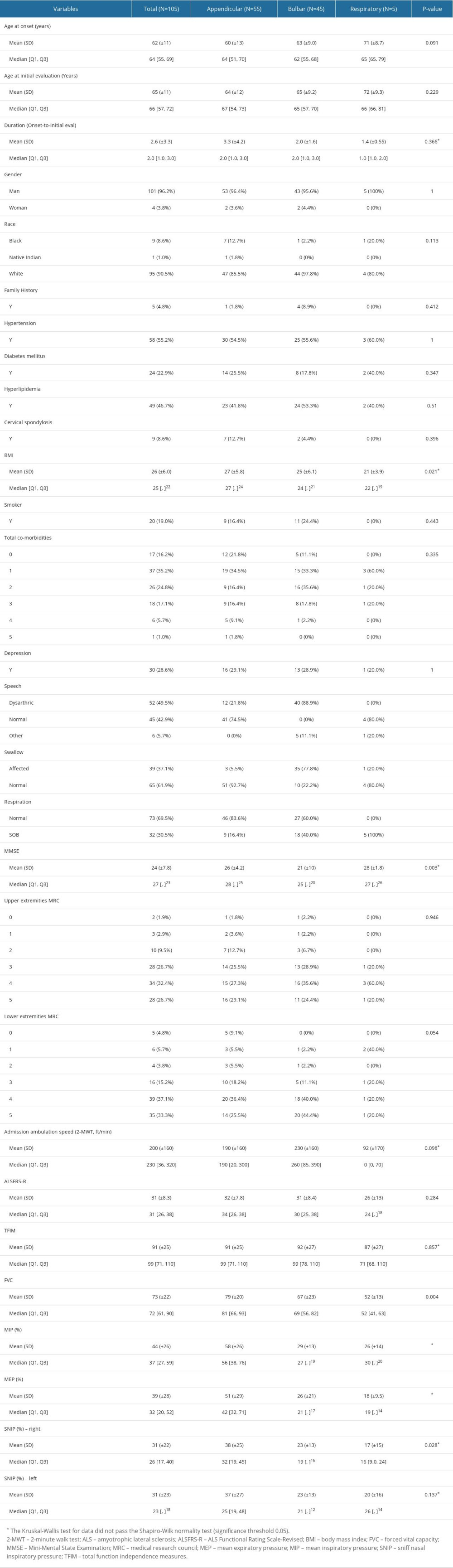
In Press
20 Mar 2024 : Clinical Research
Prevalence and Management of Chronic Pain, Including Neuropathic Pain, in Dialysis Patients with End-Stage ...Med Sci Monit In Press; DOI: 10.12659/MSM.943808
15 Mar 2024 : Clinical Research
Impact of Cluster Nursing Intervention on ICU Patients' Psychological Well-Being and Complications Associat...Med Sci Monit In Press; DOI: 10.12659/MSM.942855
26 Mar 2024 : Clinical Research
New Computerized Planning Algorithm and Clinical Testing of Optimized Nuss Bar Design for Patients with Pec...Med Sci Monit In Press; DOI: 10.12659/MSM.943705
07 May 2024 : Clinical Research
Treatment of AVN-Induced Proximal Pole Scaphoid Nonunion Using a Fifth and Fourth Extensor Compartmental Ar...Med Sci Monit In Press; DOI: 10.12659/MSM.944553
Most Viewed Current Articles
17 Jan 2024 : Review article
Vaccination Guidelines for Pregnant Women: Addressing COVID-19 and the Omicron VariantDOI :10.12659/MSM.942799
Med Sci Monit 2024; 30:e942799
14 Dec 2022 : Clinical Research
Prevalence and Variability of Allergen-Specific Immunoglobulin E in Patients with Elevated Tryptase LevelsDOI :10.12659/MSM.937990
Med Sci Monit 2022; 28:e937990
16 May 2023 : Clinical Research
Electrophysiological Testing for an Auditory Processing Disorder and Reading Performance in 54 School Stude...DOI :10.12659/MSM.940387
Med Sci Monit 2023; 29:e940387
01 Jan 2022 : Editorial
Editorial: Current Status of Oral Antiviral Drug Treatments for SARS-CoV-2 Infection in Non-Hospitalized Pa...DOI :10.12659/MSM.935952
Med Sci Monit 2022; 28:e935952