02 April 2024: Clinical Research
Predictive Value of Combined HbA1c and Neutrophil-to-Lymphocyte Ratio for Diabetic Peripheral Neuropathy in Type 2 Diabetes
Bo Lou1ABCDE*, Yu-Lan Hu1BCF, Zhong-Hua Jiang1CDDOI: 10.12659/MSM.942509
Med Sci Monit 2024; 30:e942509
Abstract
BACKGROUND: Diabetic peripheral neuropathy (DPN) is a prevalent complication affecting over 60% of type 2 diabetes patients. Early diagnosis is challenging, leading to irreversible impacts on quality of life. This study explores the predictive value of combining HbA1c and Neutrophil-to-Lymphocyte Ratio (NLR) for early DPN detection.
MATERIAL AND METHODS: An observational study was conducted at the First People's Hospital of Linping District, Hangzhou spanning from May 2019 to July 2020. Data on sex, age, biochemical measurements were collected from electronic medical records and analyzed. Employing multivariate logistic regression analysis, we sought to comprehend the factors influencing the development of DPN. To assess the predictive value of individual and combined testing for DPN, a receiver operating characteristic (ROC) curve was plotted. The data analysis was executed using R software (Version: 4.1.0).
RESULTS: The univariate and multivariate logistic regression analysis identified the level of glycated hemoglobin (HbA1C) (OR=1.94, 95% CI: 1.27-3.14) and neutrophil-to-lymphocyte ratio (NLR) (OR=4.60, 95% CI: 1.15-22.62, P=0.04) as significant risk factors for the development of DPN. Receiver operating characteristic (ROC) curve analysis demonstrated that HbA1c, NLR, and their combined detection exhibited high sensitivity in predicting the development of DPN (71.60%, 90.00%, and 97.2%, respectively), with moderate specificity (63.8%, 45.00%, and 50.00%, respectively). The area under the curve (AUC) for these predictors was 0.703, 0.661, and 0.733, respectively.
CONCLUSIONS: HbA1c and NLR emerge as noteworthy risk indicators associated with the manifestation of DPN in patients with type 2 diabetes. The combined detection of HbA1c and NLR exhibits a heightened predictive value for the development of DPN.
Keywords: Diabetes Mellitus, Hemoglobin A1c Protein, Human, Hereditary Motor And Sensory Neuropathy VI
Introduction
Diabetic neuropathy represents a prevalent chronic complication associated with diabetes, and among its various forms, diabetic peripheral neuropathy (DPN) stands out as the most frequently encountered type [1]. It is estimated that more than 60% of patients with type 2 diabetes concurrently experience DPN [2,3]. DPN manifests with a diverse range of clinical symptoms, primarily affecting the nerve endings. However, early clinical symptoms and signs of DPN may not be immediately apparent, posing challenges in achieving a diagnosis based solely on clinical manifestations [4].
As the disease advances, the emergence of symptoms and observable impairments marks the onset of DPN as an irreversible factor affecting quality of life [5]. The clinical treatment options for DPN are constrained, thus establishing it as a predominant factor leading to fatal or debilitating outcomes, notably exemplified by diabetic foot ulcers [6]. Therefore, early prediction of DPN and timely intervention are of great significance in reducing the incidence of severe complications in the later stages, as well as alleviating the economic burden on families and society [7]. Presently, within clinical practice, early diagnosis of DPN depends on a variety of neurophysiological examination techniques. However, the requirement for specialized equipment and professional expertise poses challenges to widespread adoption. Furthermore, these techniques may not identify shallow sensory nerve lesions lacking myelin sheath involvement [8].
Given the limited responsiveness of neurophysiology biomarkers in slowly progressive neuropathies, the identification of various blood biomarkers has been identified as a key goal for improving prediction and assessment of DPN [9]. Glycated hemoglobin (HbA1c) is a combination product of hemoglobin and blood glucose within red blood cells in the human bloodstream. The findings of a retrospective cohort study conducted by Cheng et al [10] revealed that HbA1c was a significant predictive factor for the occurrence of DPN. Neutrophil-to-lymphocyte ratio (NLR), an indicator of inflammation in the body, is closely associated with cancer, cardiovascular diseases, and microvascular complications in diabetes [11,12]. However, the predictive value of combined detection of HbA1c and NLR for DPN has been relatively overlooked in clinical practice. Therefore, in the present study, we conducted a retrospective analysis of risk indicators associated with the development of DPN, and explored the potential of combined risk indicator detection in predicting DPN.
Material and Methods
STUDY PARTICIPANTS:
The sample size calculation was performed using PASS software with the following parameters: Alternative Hypothesis - Two-Sided, Power – 0.9, Alpha – 0.05. The result of this calculation indicated that a minimum sample size of 44 was required for the binary logistic regression analysis. Considering the volume of cases treated in our institution, we opted to set the study sample size at 50 cases. Therefore, 100 patients diagnosed with type 2 diabetes who were admitted from May 2019 to July 2020, as well as 100 healthy individuals who underwent health check-ups during the same period at the hospital, were selected as study participants. Participants with type 2 diabetes were subsequently divided into 2 groups: the type-2 diabetes group without DPN (T2DM; n=50) and the type-2 diabetes group with DPM (T2DM+DPN; n=50).
The diagnosis of type 2 diabetes and DPN was conducted following the guidelines released by the Chinese Society of Diabetes, in 2017, and the Chinese Society of Neurology, in 2013 [13,14]. The fundamental diagnostic criteria for DPN encompass: (1) a well-defined history of diabetes; (2) manifestation of neuropathy either at the time of confirmed diabetes diagnosis or subsequently; and (3) clinical presentation of neuropathic symptoms. Examples of neuropathic symptoms include pain, numbness, and abnormal sensations, including any single abnormality in the 5 diagnostic tests (ankle reflex, vibration sensation, pressure sensation, pinprick sensation, or temperature sensation). In the absence of clinical symptoms, the presence of any 2 abnormalities in the 5 tests is sufficient for diagnosis. Patients with peripheral neuropathy caused by other etiologies, such as drug-induced neurotoxicity (resulting from chemotherapeutic drugs), vitamin B12 deficiency, cervical or lumbar spine disorders (including compression, stenosis, or degenerative changes), cerebral infarction, chronic inflammatory demyelinating polyneuropathy, infectious polyneuropathy, vasculitis, and other related conditions, were excluded from the present study. Moreover, individuals experiencing severe dysfunction in cardiac, hepatic, or renal function; those diagnosed with acute inflammatory or immune system diseases, malignancies, or hematological disorders; and individuals undergoing treatment with glucocorticoids or immunomodulatory therapies – conditions that may lead to notable fluctuations in biochemical measurements – were also excluded from the current study. The present study was conducted following the ethical guidelines outlined in the 1975 Declaration of Helsinki and received approval from the Ethics Committee of First People’s Hospital of Linping District, Hangzhou (No. 2021021).
BIOCHEMICAL MEASUREMENTS:
Fasting peripheral venous blood samples of 5 mL were collected from the study participants in the morning. Serum was obtained by centrifugation at 3000 rpm for 15 minutes at 4°C to measure the total cholesterol (TC), triglycerides (TG), high density lipoprotein cholesterol (HDL-C), low-density lipoprotein cholesterol (LDL-C), fasting blood glucose, fasting insulin (Fins), HbA1c, 24-hour urinary albumin excretion rate (24-h UAER), cystatin C (Cys-C), and NLR. The homeostasis model assessment of insulin resistance (HOMA-IR) and homeostasis model assessment of β-cell function (HOMA-β) were calculated to evaluate insulin resistance and β-cell function.
STATISTICAL ANALYSIS:
Statistical analysis of the data was performed using R software (Version: 4.1.0), adhering to rigorous methodologies. To assess the normality of the data, the Shapiro-Wilk test was employed. The biochemical indicators were represented by the median, along with the interquartile range [25th–75th percentile]. Initially, a Kruskal-Wallis test was conducted for each indicator, and subsequently, significant differences between groups were assessed using pairwise comparisons through the Mann-Whitney U test. To identify independent predictors of DPN occurrence, multivariate logistic regression analysis was executed, ensuring a robust exploration of potential factors influencing the outcome. The predictive value of HbA1c and NLR for the development of DPN was evaluated by plotting the receiver operating characteristic (ROC) curve. A
Results
BASELINE CHARACTERISTICS OF THE PARTICIPANTS:
The T2DM+DPN group consisted of 28 male and 22 female patients, and the T2DM group also had 28 male and 22 female patients. In the healthy control group, there were 61 male and 39 female patients. The age of the patients in the T2DM+DPN group ranged from 26 to 70 years, with a mean age±standard deviation of 55.70±9.55 years. For the patients in the T2DM group, their ages ranged from 34 to 70 years, with a mean age ± standard deviation of 52.66±10.38 years. In the healthy control group, the age range was from 30 to 73 years, with a mean age±standard deviation of 49.70±10.62 years.
PREDICTIVE FACTORS FOR DPN DEVELOPMENT:
Comparison of the biochemical indicators among the 3 groups showed that study participants with type 2 diabetes demonstrated higher levels of TC, TG, LDL-C, HbA1c, FBG, 24-h UAER, Cys-C, and NLR, while exhibiting lower levels of HDL-C, Fins, HOMA-IR, and HOMA-β, in comparison to healthy individuals (Table 1). Furthermore, the univariate logistic regression model revealed associations between the development of DPN and the following biochemical measurements: HDL-C (OR=0.27, 95% CI: 0.07–0.94, P=0.04), HbA1c (OR=1.92, 95% CI: 1.35–2.88, P<0.01), HOMA-β (OR=0.96, 95% CI: 0.92–0.99, P=0.05), and NLR (OR=6.30, 95% CI: 1.67–29.43, P=0.01) (Table 2). The pairwise comparisons of these 4 biochemical measurements among the 3 groups are illustrated in Figure 1.
To further identify the potential predictive factors influencing the development of DPN in patients with type 2 diabetes, multivariate logistic regression models were constructed with the occurrence of DPN as the dependent variable and the 4 biochemical measurements as independent variables. On the multivariate (Table 3) analysis, elevated levels of HbA1c (OR=1.94, 95% CI: 1.27–3.14, P<0.01) and NLR (OR=4.60, 95% CI: 1.15–22.62, P=0.04) were significant risk factors for the development of DPN.
PREDICTIVE VALUES OF HBA1C AND NLR FOR DPN DEVELOPMENT:
Based on the clinical diagnosis of DPN, ROC curves were plotted with the occurrence of DPN as the outcome variable, and HbA1c and NLR as the diagnostic variables. The results indicated that HbA1c had the highest specificity for predicting the development of DPN. However, the combination of HbA1c and NLR exhibited the highest area under the curve (AUC) and sensitivity in predicting the development of DPN (Table 4). The optimal cutoff values for HbA1c and NLR, determined by the point on the ROC curve closest to the upper left corner, were 7.55 and 2.64, respectively (Figure 2).
Discussion
The primary finding of this study underscored that both HbA1c and NLR levels were significant risk factors for the development of DPN. These findings are consistent with the outcomes reported in the meta-analysis conducted by Liu et al [15] and retrospective study performed by Demirdal et al [16]. In the progression of DPN, the elevated levels of blood glucose associated with this condition can lead to damage or impairment of various tissues and organs [17,18]. DPN is acknowledged as among the more prevalent and severe complications of type 2 diabetes. In addition to persistent high blood glucose, fluctuations in blood glucose levels also contribute as a risk factor for the development of DPN [19]. These fluctuations can have detrimental effects on the occurrence and progression of chronic complications in diabetes by triggering several mechanisms. These mechanisms include the activation of oxidative stress pathways, the impairment of vascular endothelial cells, the promotion of cell apoptosis, DNA oxidative damage, and the exacerbation of chronic inflammatory states [20,21].
HbA1c plays a crucial role as a widely used biomarker in clinical practice for assessing blood glucose control. It is formed through the non-enzymatic binding of blood glucose with hemoglobin inside red blood cells, which is independent of fasting status and insulin treatment and can reflect the average blood glucose level in the 6–10 weeks prior to testing. This binding alters the oxygen affinity of red blood cells, potentially causing oxidative stress and inflammatory damage [22]. Numerous studies have consistently demonstrated a correlation between elevated HbA1c levels in patients with type 2 diabetes and an increased risk of various complications [23,24]. These findings highlight the importance of maintaining optimal HbA1c levels as a key factor in the effective management and prevention of diabetic complications. The results of the present study demonstrated that HbA1c was a significant risk factor for the development of DPN. In a cross-sectional study conducted by Hunaifi et al [25], the findings indicated a correlation between HbA1c levels and the severity of diabetic neuropathy in individuals with type 2 diabetes. These studies provide valuable evidence supporting the role of HbA1c as a marker for assessing the severity and risk of DPN in patients with type 2 diabetes.
In addition, our study also identified another significant risk factor associated with the development of DPN, which is NLR. NLR has emerged as a valuable indicator of inflammatory response, characterized by an elevation in neutrophil count and/or a decrease in lymphocyte count [26]. NLR testing is not only straightforward and cost-effective but also less susceptible to the influence of pathological and physiological conditions, offering greater stability and specificity. Neutrophils and lymphocytes are 2 primary subtypes of leukocytes. Elevated levels of neutrophils release a significant amount of inflammatory mediators, which can result in endothelial damage and impairments in microcirculation [27]. Conversely, inflammatory factors can prompt an increase in neutrophil numbers, resulting in a chronic, low-grade state of inflammation that negatively impacts the nervous system [28]. Lymphocytes and neutrophils have distinct roles in the body’s inflammatory response, playing regulatory and protective roles during the occurrence and progression of inflammation [29]. Collectively, these studies highlight the importance of timely and effective early clinical intervention aimed at addressing the chronic inflammatory state in the progression of T2DM to help prevent the onset of DPN. The retrospective study conducted by Liu et al [11] and the observational study by Chen et al [30] both demonstrated a significant correlation between NLR and DPN. The results of the present study indicated that NLR was predictive of the development of DPN with a high sensitivity, which is consistent with the results reported by Demirdal et al [16]. In addition, the findings of the present study demonstrated that the simultaneous assessment of HbA1c and NLR resulted in the highest AUC and sensitivity for predicting the onset of DPN.
The present study has certain limitations. Firstly, it is a single-center study with a relatively small sample size, and expanding to include more centers and a larger sample would enhance the robustness and generalizability of our findings. Additionally, certain biomarkers have limitations and may not capture all aspects of DPN. Thus, future research should consider incorporating a broader array of evaluation biomarkers to provide a more comprehensive understanding of DPN. On a positive note, this study contributes significantly by pioneering the use of a combination of HbA1c and NLR for assessing the development of DPN in patients with type 2 diabetes, marking a novel approach in this field.
Conclusions
Taken together, this study evaluated predictive factors for the development of DPN and specifically investigated the predictive value of HbA1c combined with NLR in the onset of DPN. HbA1c and NLR are significant risk indicators for the presence of DPN in patients with type 2 diabetes. Furthermore, the combined use of HbA1c and NLR has a higher predictive value for the development of DPN than either biomarker alone. However, it is important to note that this study has limitations as it is a single-center, small observational study. Therefore, it is crucial for future research to prioritize large-sample, multicenter studies to further investigate this topic.
Figures
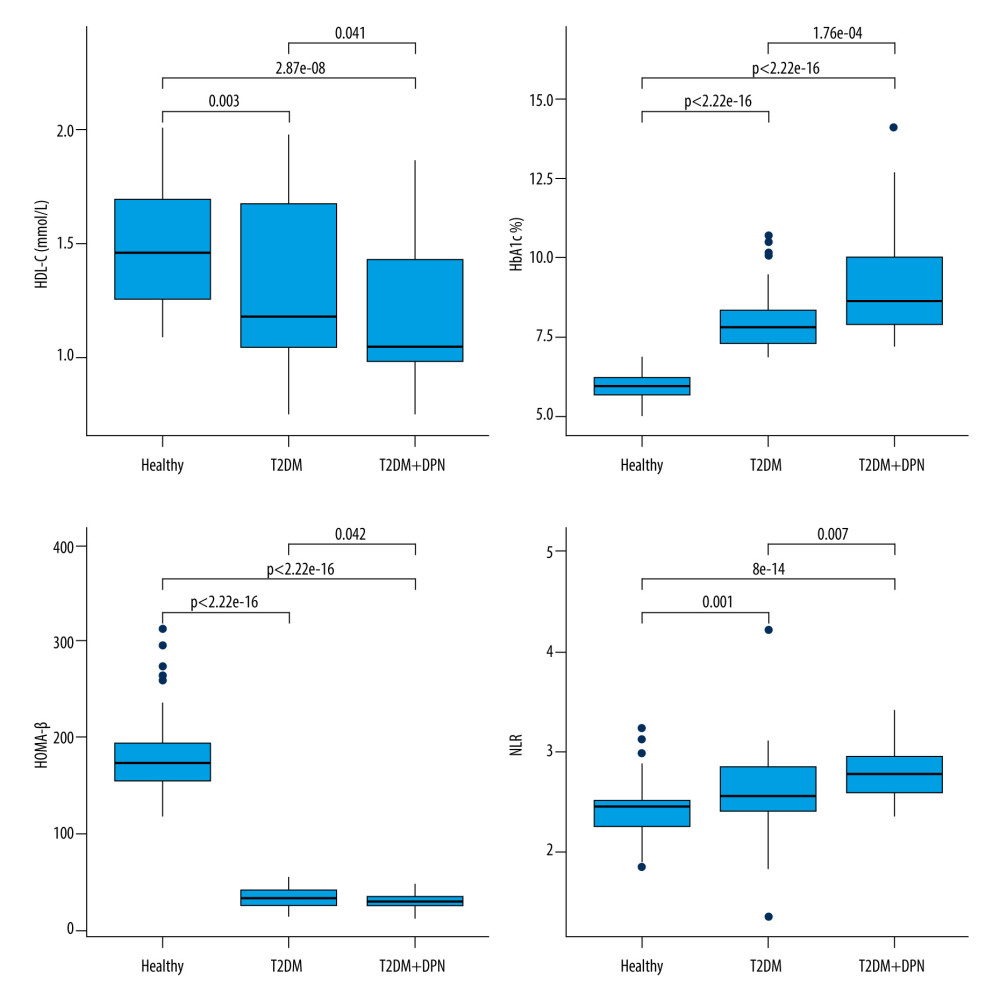
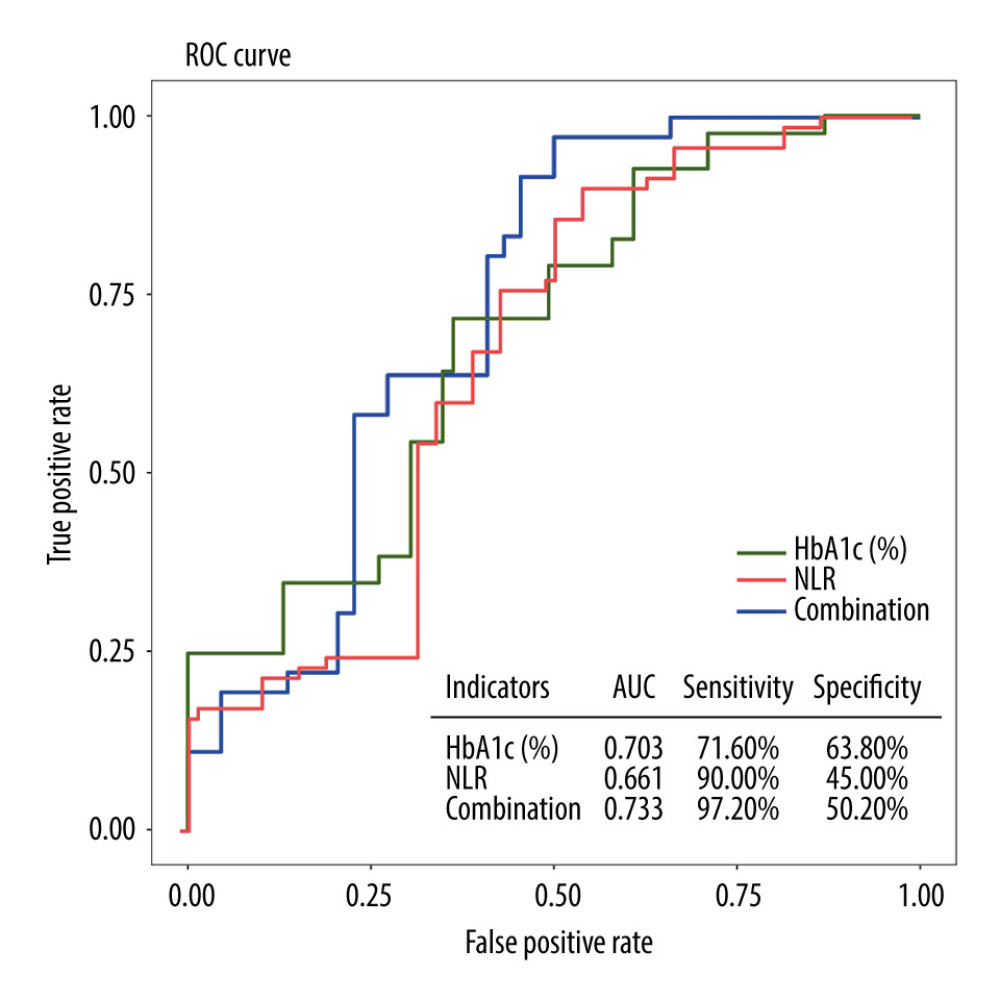
Tables
Table 1. Comparison of biochemical indicators among the 3 groups by Kruskal-Wallis test.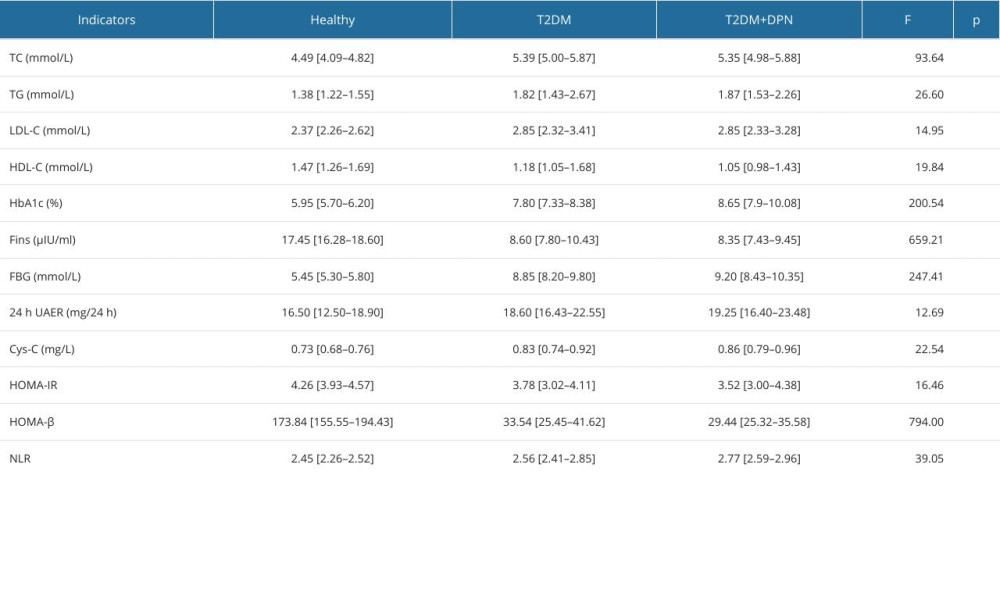
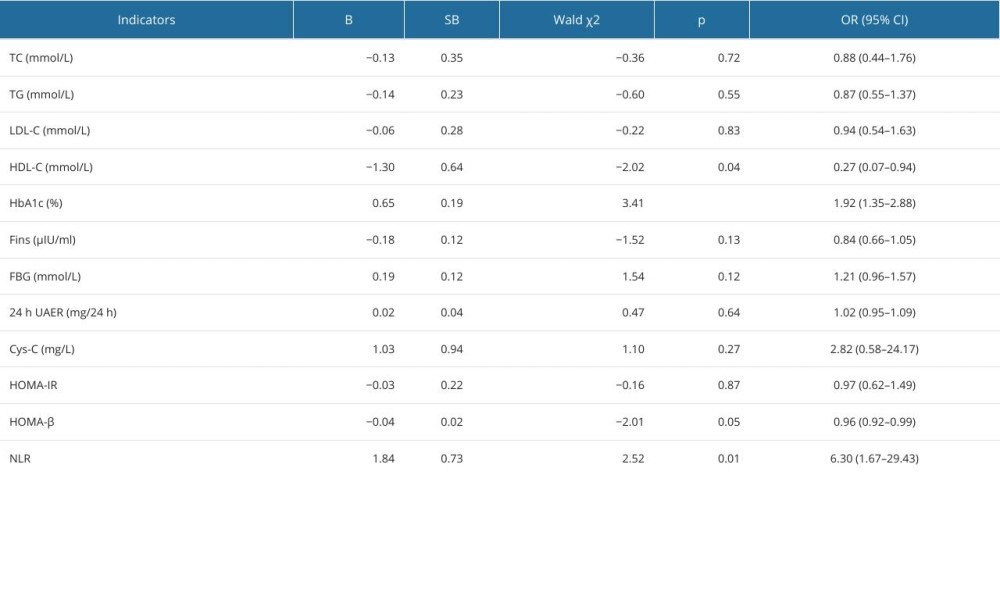
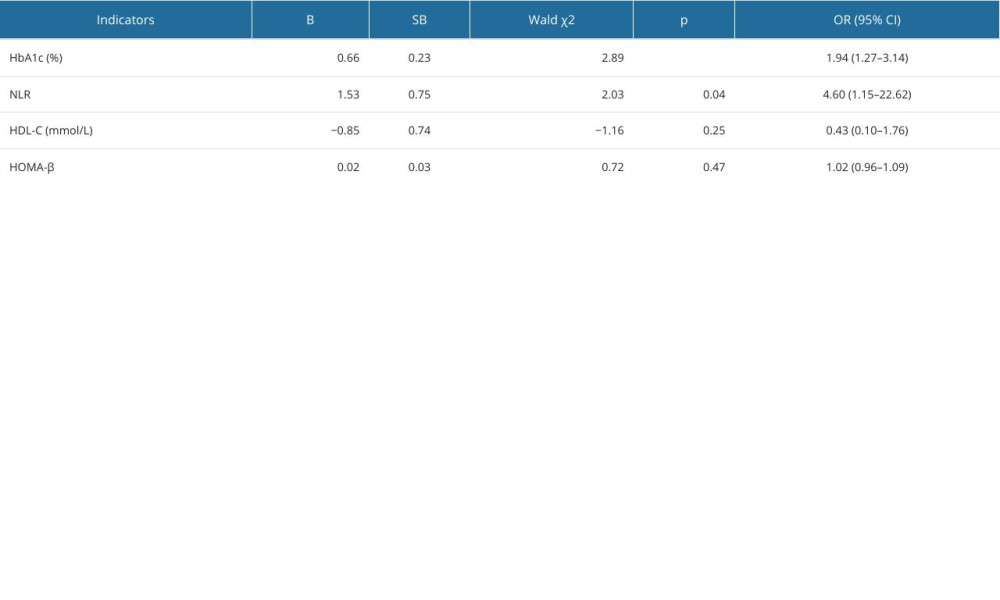
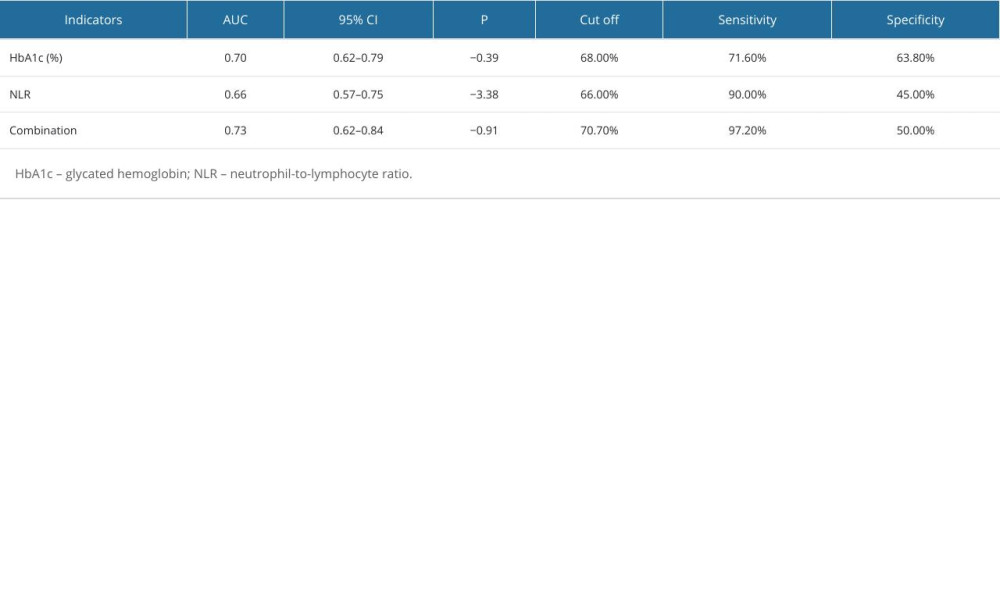
References
1. Zakin E, Abrams R, Simpson DM, Diabetic neuropathy: Semin Neurol, 2019; 39(5); 560-69
2. Wang W, Ji Q, Ran X, Prevalence and risk factors of diabetic peripheral neuropathy: A population-based cross-sectional study in China: Diabetes Metab Res Rev, 2023; 39(8); e3702
3. Farias ALd, Alves da Silva AS, Tavares VB, Amazon amandaba-prevalence, risk factors and self-care perception associated with diabetic peripheral neuropathy in patients with type 2 diabetes: A cross-sectional study: Healthcare, 2023; 11(4); 518
4. Callaghan BC, Cheng HT, Stables CL, Diabetic neuropathy: Clinical manifestations and current treatments: Lancet Neurol, 2012; 11(6); 521-34
5. Carmichael J, Fadavi H, Ishibashi F, Advances in screening, early diagnosis and accurate staging of diabetic neuropathy: Front Endocrinol, 2021; 12; 671257
6. Khdour MR, Treatment of diabetic peripheral neuropathy: A review: J Pharm Pharmacol, 2020; 72(7); 863-72
7. Wu B, Niu Z, Hu F, Study on risk factors of peripheral neuropathy in type 2 diabetes mellitus and establishment of prediction model: Diabetes Metab J, 2021; 45(4); 526-38
8. Burgess J, Frank B, Marshall A, Early detection of diabetic peripheral neuropathy: A focus on small nerve fibres: Diagnostics, 2021; 11(2); 165
9. Rossor AM, Reilly MM, Blood biomarkers of peripheral neuropathy: Acta Neurol Scand, 2022; 146(4); 325-31
10. Cheng Y, Cao W, Zhang J, Determinants of diabetic peripheral neuropathy and their clinical significance: A retrospective cohort study: Front Endocrinol, 2022; 13; 934020
11. Liu S, Zheng H, Zhu X, Neutrophil-to-lymphocyte ratio is associated with diabetic peripheral neuropathy in type 2 diabetes patients: Diabetes Res Clin Pract, 2017; 130; 90-97
12. Wu L, Zou S, Wang C, Neutrophil-to-lymphocyte and platelet-to-lymphocyte ratio in Chinese Han population from Chaoshan region in South China: BMC Cardiovasc Disord, 2019; 19(1); 125
13. Jia W, Weng J, Zhu D, Standards of medical care for type 2 diabetes in China 2019: Diabetes Metab Res Rev, 2019; 35(6); e3158
14. Dong L, He W, Zhang R, Artificial intelligence for screening of multiple retinal and optic nerve diseases: JAMA Netw Open, 2022; 5(5); e229960
15. Liu X, Xu Y, An M, The risk factors for diabetic peripheral neuropathy: A meta-analysis: PLoS One, 2019; 14(2); e0212574
16. Demirdal T, Sen P, The significance of neutrophil-lymphocyte ratio, platelet-lymphocyte ratio and lymphocyte-monocyte ratio in predicting peripheral arterial disease, peripheral neuropathy, osteomyelitis and amputation in diabetic foot infection: Diabetes Res Clin Pract, 2018; 144; 118-25
17. Tabara Y, Takahashi Y, Setoh K, Synergistic association of elevated serum free fatty acid and glucose levels with large arterial stiffness in a general population: The Nagahama Study: Metabolism, 2016; 65(1); 66-72
18. Poznyak AV, Litvinova L, Poggio P, Effect of glucose levels on cardiovascular risk: Cells, 2022; 11(19); 3034
19. Yapanis M, James S, Craig ME, Complications of diabetes and metrics of glycemic management derived from continuous glucose monitoring: J Clin Endocrinol Metab, 2022; 107(6); e2221-e36
20. Yan T, Zhang Z, Li D, NGF receptors and PI3K/AKT pathway involved in glucose fluctuation-induced damage to neurons and α-lipoic acid treatment: BMC Neurosci, 2020; 21(1); 38
21. Xin H, Fu Y, Feng M, Altered intrinsic brain activity related to neurologic and motor dysfunction in diabetic peripheral neuropathy patients: J Clin Endocrinol Metab, 2023; 108(4); 802-11
22. Paudel YN, Ali MR, Bawa S, Evaluation of 4-methyl-2-[(2-methylbenzyl) amino]-1,3-thiazole-5-carboxylic acid against hyperglycemia, insulin sensitivity, and oxidative stress-induced inflammatory responses and β-cell damage in the pancreas of streptozotocin-induced diabetic rats: Hum Exp Toxicol, 2018; 37(2); 163-74
23. Mencke R, van der Vaart A, Pasch A, Serum calcification propensity is associated with HbA1c in type 2 diabetes mellitus: BMJ Open Diabetes Res Care, 2021; 9(1); e002016
24. Qureshi A, Haque Z, Bokhari SAH, Evaluation of HbA1c in type-2 diabetes mellitus patients with periodontitis: Preliminary findings of three-arm clinical trial: JPMA J Pak Med Assoc, 2020; 70(8); 1350-56
25. Hunaifi I, Agustriadi I, Asmara IGY, The correlation between HbA1c and neuropathy disability score in type 2 diabetes: Acta Med Indones, 2021; 53(2); 164-68
26. Zahorec R, Neutrophil-to-lymphocyte ratio, past, present and future perspectives: Bratisl Lek Listy, 2021; 122(7); 474-88
27. Barkaway A, Rolas L, Joulia R, Age-related changes in the local milieu of inflamed tissues cause aberrant neutrophil trafficking and subsequent remote organ damage: Immunity, 2021; 54(7); 1494-1510.e7
28. Chakraborty S, Tabrizi Z, Bhatt NN, A brief overview of neutrophils in neurological diseases: Biomolecules, 2023; 13(5); 743
29. Cronkite DA, Strutt TM, The Regulation of inflammation by innate and adaptive lymphocytes: J Immunol Res, 2018; 2018 1467538
30. Chen M, Zhu Y, Wang J, The predictive value of neutrophil-to-lymphocyte ratio and platelet-to-lymphocyte ratio levels of diabetic peripheral neuropathy: J Pain Res, 2021; 14; 2049-58
Figures
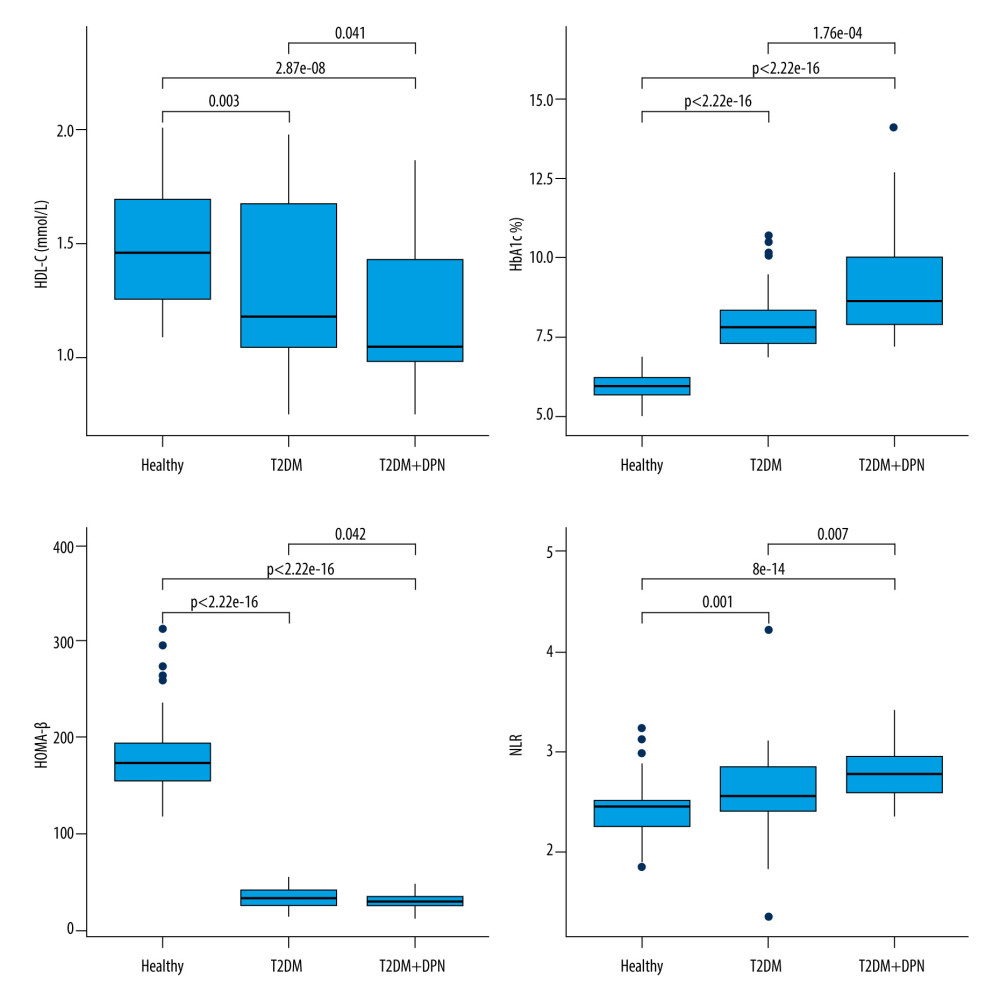
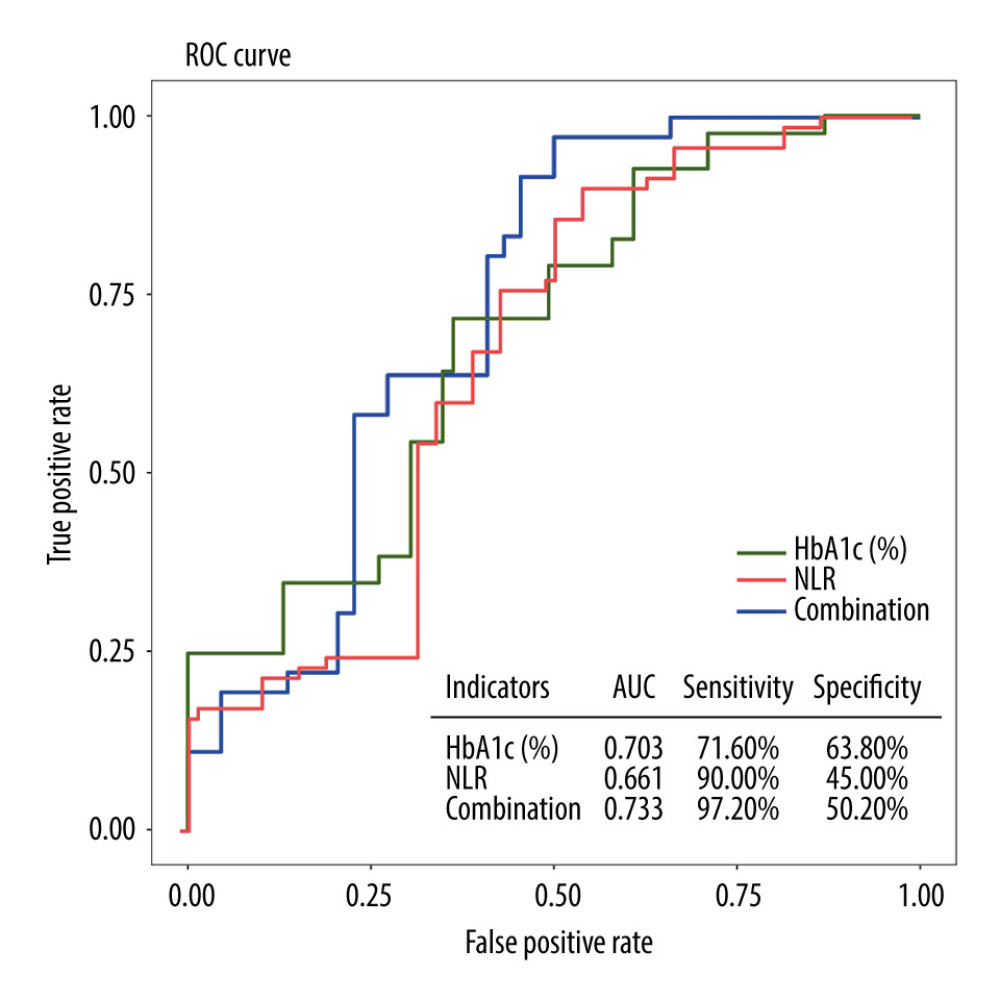
Tables
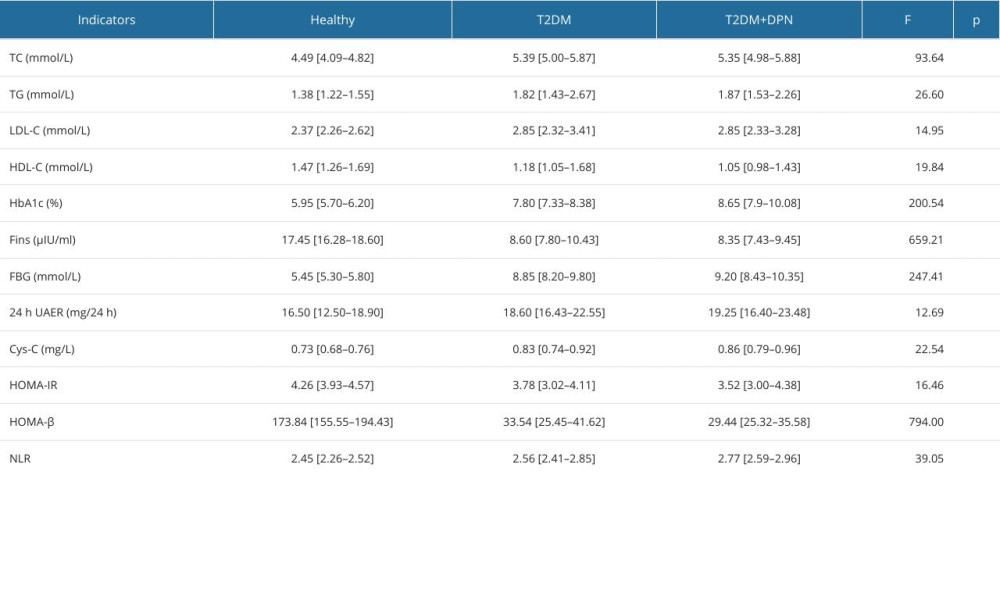
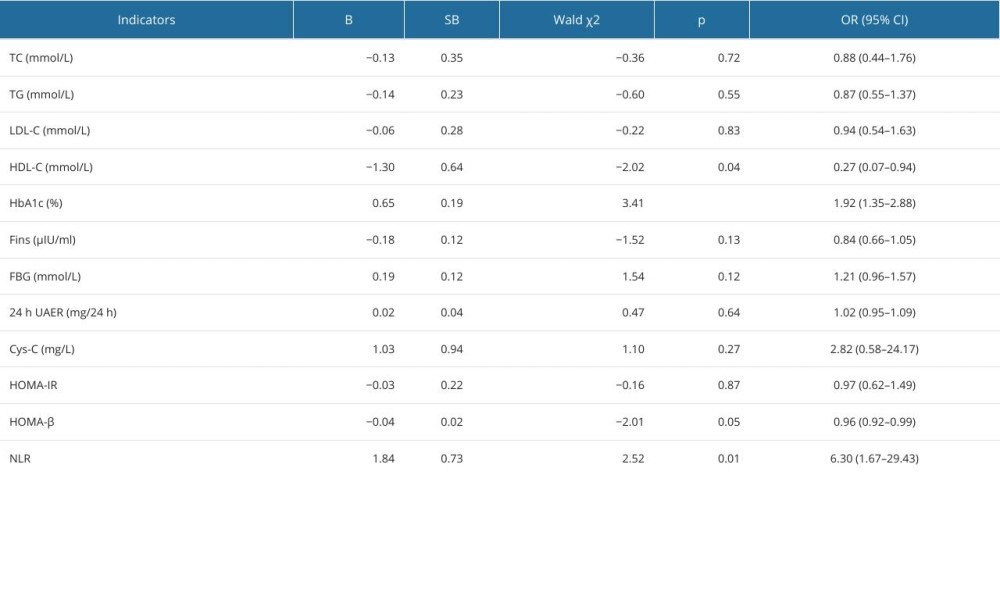
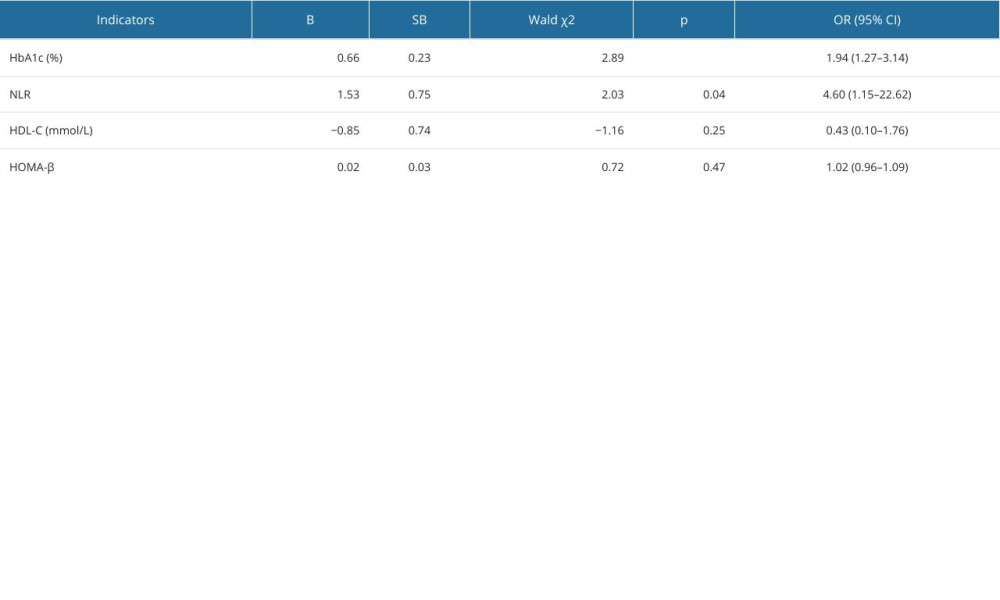
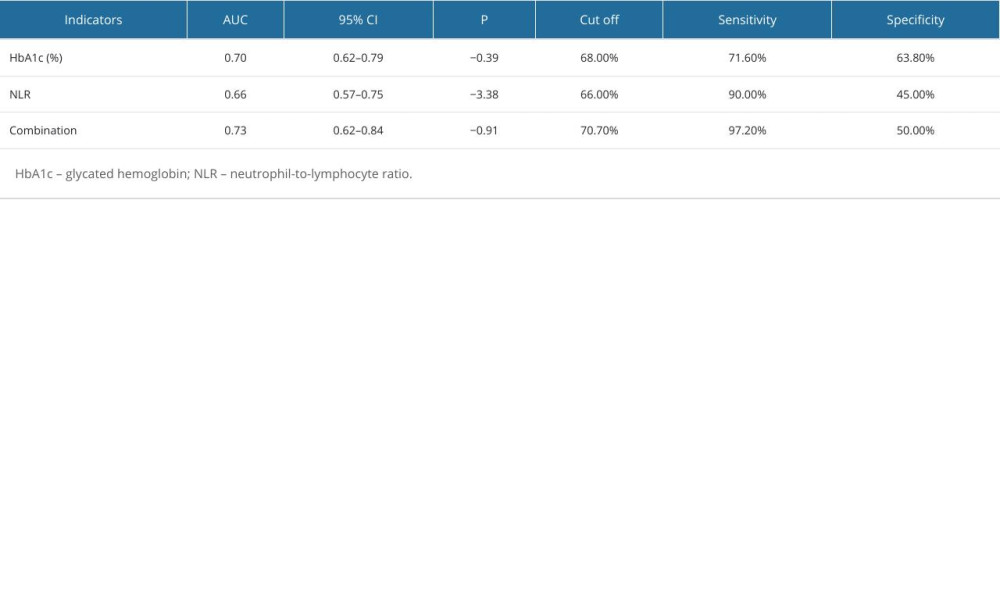
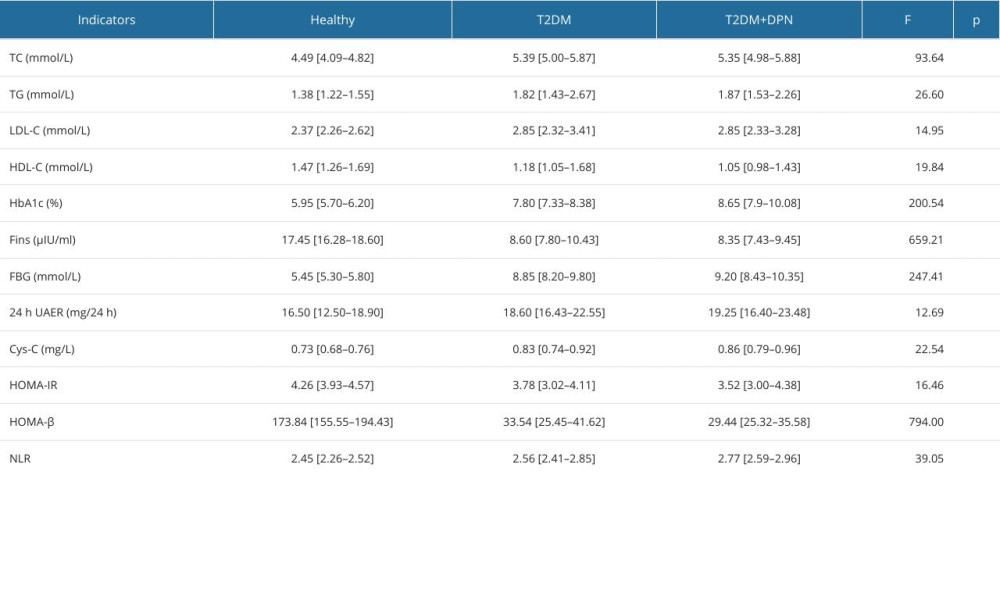
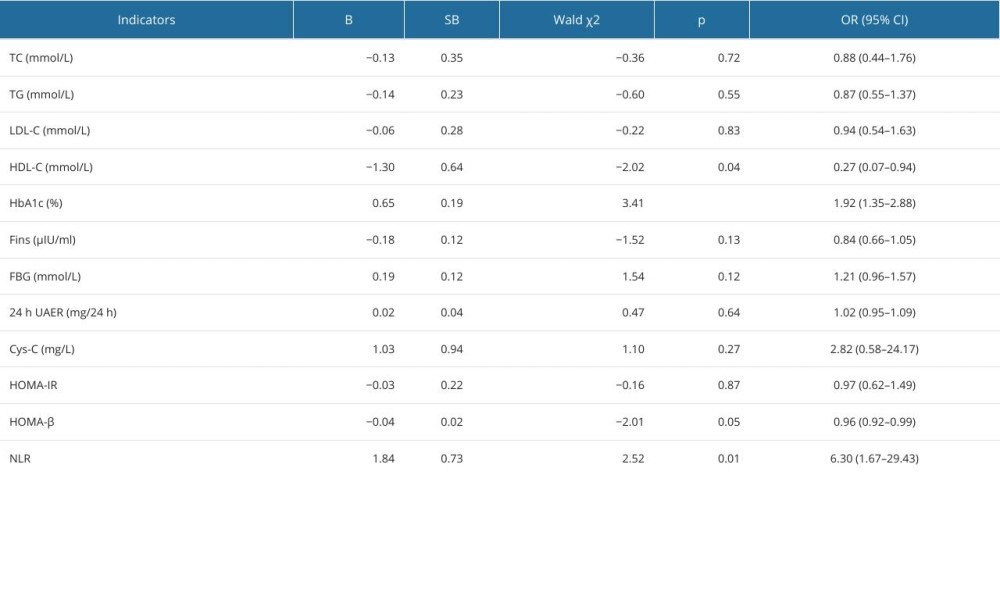
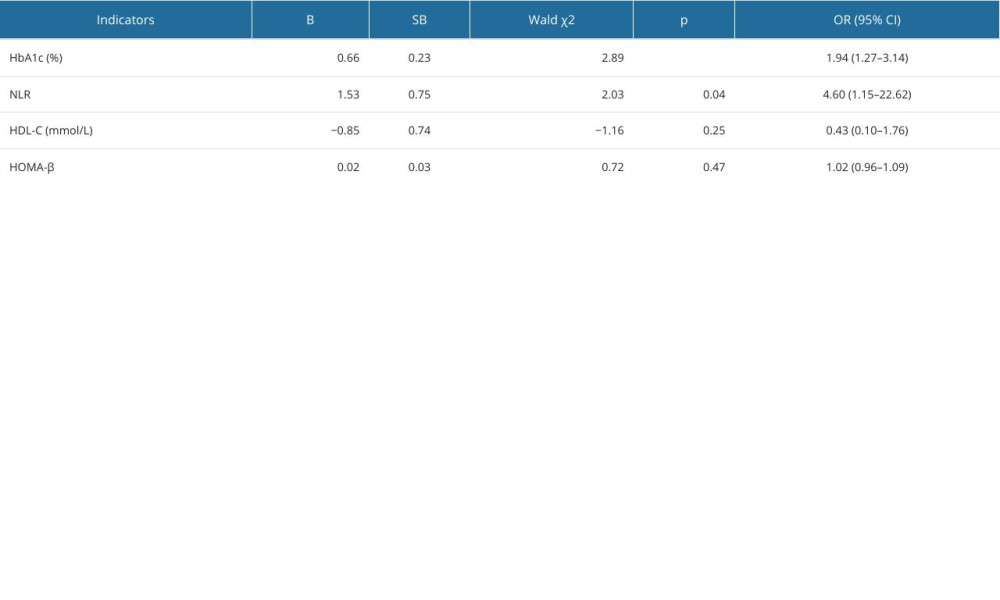
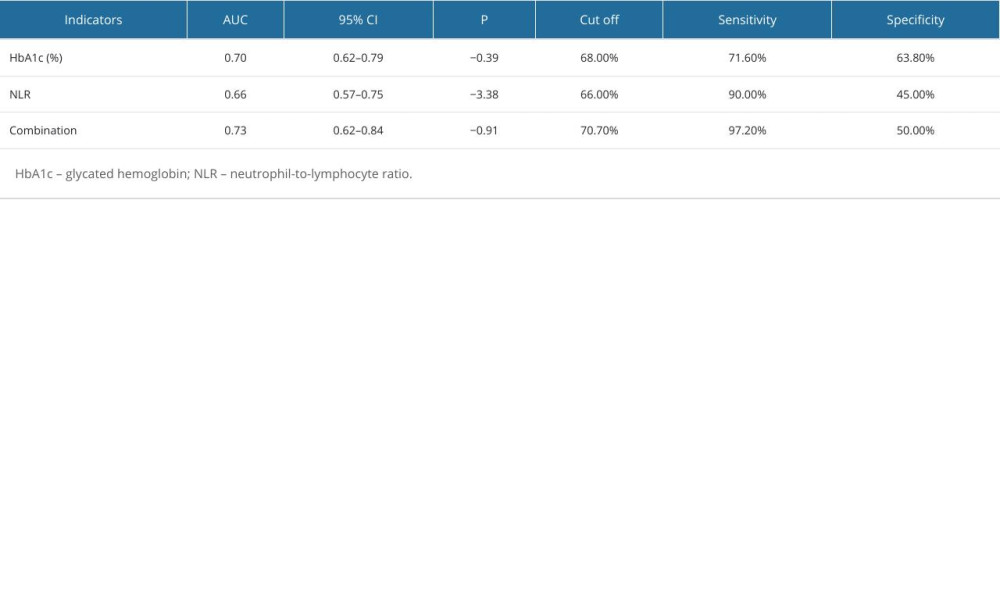
In Press
08 Mar 2024 : Animal Research
Modification of Experimental Model of Necrotizing Enterocolitis (NEC) in Rat Pups by Single Exposure to Hyp...Med Sci Monit In Press; DOI: 10.12659/MSM.943443
18 Apr 2024 : Clinical Research
Comparative Analysis of Open and Closed Sphincterotomy for the Treatment of Chronic Anal Fissure: Safety an...Med Sci Monit In Press; DOI: 10.12659/MSM.944127
08 Mar 2024 : Laboratory Research
Evaluation of Retentive Strength of 50 Endodontically-Treated Single-Rooted Mandibular Second Premolars Res...Med Sci Monit In Press; DOI: 10.12659/MSM.944110
11 Mar 2024 : Clinical Research
Comparison of Effects of Sugammadex and Neostigmine on Postoperative Neuromuscular Blockade Recovery in Pat...Med Sci Monit In Press; DOI: 10.12659/MSM.942773
Most Viewed Current Articles
17 Jan 2024 : Review article
Vaccination Guidelines for Pregnant Women: Addressing COVID-19 and the Omicron VariantDOI :10.12659/MSM.942799
Med Sci Monit 2024; 30:e942799
14 Dec 2022 : Clinical Research
Prevalence and Variability of Allergen-Specific Immunoglobulin E in Patients with Elevated Tryptase LevelsDOI :10.12659/MSM.937990
Med Sci Monit 2022; 28:e937990
16 May 2023 : Clinical Research
Electrophysiological Testing for an Auditory Processing Disorder and Reading Performance in 54 School Stude...DOI :10.12659/MSM.940387
Med Sci Monit 2023; 29:e940387
01 Jan 2022 : Editorial
Editorial: Current Status of Oral Antiviral Drug Treatments for SARS-CoV-2 Infection in Non-Hospitalized Pa...DOI :10.12659/MSM.935952
Med Sci Monit 2022; 28:e935952