15 May 2023: Clinical Research
Accuracy of Self-Injection Locking Radar System for Vital Signs Detection During the COVID-19 Pandemic at a Hospital in Taiwan: Measuring Vital Signs Accurately with SIL Radar for Hospital Healthcare
Shiow-Jyu Tzou12AB, I-Hung Chen23AB, Tian-Huei Chu12CDE, De-Ming Chian4ABC, Fu-Kang Wang5AD, Yung-Kuo Lee12CDEFG*, Chou-Yuan Ko26ABCDGDOI: 10.12659/MSM.939949
Med Sci Monit 2023; 29:e939949
Abstract
BACKGROUND: Self-injection locking (SIL) radar uses continuous-wave radar and an injection-locked oscillator-based frequency discriminator that receives and demodulates radar signals remotely to monitor vital signs. This study aimed to compare SIL radar with traditional electrocardiogram (ECG) measurements to monitor respiratory rate (RR) and heartbeat rate (HR) during the COVID-19 pandemic at a single hospital in Taiwan.
MATERIAL AND METHODS: We recruited 31 hospital staff members (16 males and 15 females) for respiratory rates (RR) and heartbeat rates (HR) detection. Data acquisition with the SIL radar and traditional ECG was performed simultaneously, and the accuracy of the measurements was evaluated using Bland-Altman analysis.
RESULTS: To analyze the results, participates were divided into 2 groups (individual subject and multiple subjects) by gender (male and female), or 4 groups (underweight, normal weight, overweight, and obesity) by body mass index (BMI). The results were analyzed using mean bias errors (MBE) and limits of agreement (LOA) with a 95% confidence interval. Bland-Altman plots were utilized to illustrate the difference between the SIL radar and ECG monitor. In all BMI groups, results of RR were more accurate than HR, with a smaller MBE. Furthermore, RR and HR measurements of the male groups were more accurate than those of the female groups.
CONCLUSIONS: We demonstrated that non-contact SIL radar could be used to accurately measure HR and RR for hospital healthcare during the COVID-19 pandemic.
Keywords: Data Accuracy, Heart Rate, Radar, Respiratory Rate, vital signs, Male, Humans, Female, Signal Processing, Computer-Assisted, Taiwan, Pandemics, COVID-19, Hospitals, Algorithms, Monitoring, Physiologic
Background
The assessment of vital signs, such as respiratory rate (RR), heartbeat rate (HR), blood pressure, and body temperature, plays an important role in monitoring essential body functions in various medical conditions [1]. Accurate measurement for monitoring vital signs with traditional techniques requires that sensors, as in electrocardiography (ECG), be attached to a subject. However, the global COVID-19 pandemic and other infectious diseases have raised concerns regarding traditional monitoring methods in hospitals. The development of a non-contact monitoring system for hospital healthcare [2–5] is required.
SIL radar was first proposed in 2010 for vital sign detection using continuous-wave radar and an injection-locked oscillator-based frequency discriminator [6]. Non-contact self-injection locking (SIL) radar to track the RR and HR in real time, as well as high resolution in laboratory testing, produces highly accurate measurements with multiple persons [6–8]. Long-term monitoring of vital signs from a remote location using noninvasive and low-cost devices in a clinical setting is preferred [9–11]. However, highly accurate measurements of vital signs with SIL radar in hospitals need to be evaluated. We applied and evaluated the performance of 31 hospital workers using SIL radar as a remote method to assess RR and HR compared to ECG.
To reduce the risks, such as transmission and infection of the COVID-19 virus, for clinical staff, use of contactless radar to assess vital signs of isolated patients is important. Therefore, the aim of this study was to evaluate the accuracy of SIL radar for RR and HR detection in hospital participants with the Bland-Altman analysis during the COVID-19 pandemic. In the future, SIL radar contactless monitoring will be an effective solution for large-scale patient monitoring during global pandemics.
Material and Methods
Study Design
PARTICIPANTS: All participants, including clinical and non-clinical staff, were recruited from Kaohsiung Armed Forces General Hospital. Personal information stratified by gender, number of subjects, and BMI is shown in Table 1. According to the Health Promotion Administration in Taiwan, BMI <18.5, 18.5≤ BMI <24, 24≤ BMI <27, and 27≤ BMI are defined as underweight, normal weight, overweight, and obese, respectively.
ETHICS APPROVAL:
Informed consent was obtained from all study participants. All procedures were approved and all participants were monitored by the Institutional Review Board of Kaohsiung Armed Forces General Hospital Kaohsiung Armed Forces General Hospital (KAFGHIIRB 109-053) in accordance ethics standards and the 1964 Helsinki Declaration.
DATA COLLECTION FOR THE SIL RADAR AND THE ECG: A single antenna of the SIL radar [8] was placed over the headboard of the bed (Figure 1) in the hospital. The signals from the SIL radar and the ECG were collected and recorded simultaneously. The RR and HR detection data from SIL radar were separated and tracked according to a previous study [8]. ECG was measured with the SureSigns VM6 device (Philips) to derive the RR and HR as the ground truth.
STATISTICAL ANALYSIS: We collected and compared data of RR and HR from SIL radar and ECG recordings. The method comparison for the accuracy of the data of the new monitor was established by Bland-Altman analysis [12–16]. Bland-Altman analysis is the most commonly used statistical analysis for accessing accuracy of data with limits of agreement (LOA) from 2 medical devices (SIL radar and ECG) [17,18]. LOA shows a range of agreement with 95% of the differences between the 2 medical devices we used. We found highly accurate measurements on RR and HR of all participants between the 2 devices with small values of mean bias errors and LOA. All statistical analyses were performed in MedCalc (MedCalc Software, Ltd., Ostend, Belgium).
Results
HIGH ACCURACY OF RR AND HR OBTAINED BY CONTACTLESS RADAR MONITORING IN INDIVIDUAL USER GROUP:
There were 518 RR samples and 539 HR samples. For RR, Bland-Altman analysis showed that the bias of individual users was 0.9±5.7 beats/min with 95% LOA of 12 to −10.2 beats/min (Figure 2A), and the bias of multiusers was −1.3±7.4 beats/min with 95% LOA of 13.2 to −15.8 beats/min (Figure 2B). For HR, Bland-Altman analysis showed that the bias of individual user was 1.1±13.9 beats/min with 95% LOA of 28.4 to −26.1 beats/min (Figure 2C), and the bias of multiuser was −5.9±18.3 beats/min with 95% LOA 30.1 to −41.8 beats/min (Figure 2D). Estimations of RR and HR of the radar in individual user groups showed higher accuracy than in multiuser groups.
ACCURATE MEASUREMENTS OF RR AND HR OBTAINED BY CONTACTLESS RADAR MONITORING IN THE MALE GROUP:
Next, we analyzed the RR and HR data of each user and multiple users by gender. A total of 160 samples and 160 samples recorded for RR and HR from 16 male participants and a total of 139 samples and 140 samples recorded from 15 female participants were included in the analysis. The Bland-Altman plots of RR and HR showed a bias of 0.4±5.6 beats/min with 11.3 to −10.5 beats/min at 95% LOA and 0.9±11.3 beats/min with 12.9 to −14.6 beats/min at 95% LOA within single male group (Figures 3, 4). Measurements of RR and HR in a single specific male subgroup from radar were more accurate with smaller mean bias errors values than other groups.
ALL BMI GROUPS SHOWED A HIGH LEVEL OF ACCURACY FOR THE MEASUREMENT OF RR:
In the 4 BMI groups, for RR, a bias of 0.9±5.5 beats/min with 11.5 and −10.0 beats/min 95% LOA was found in the underweight group; 1.2±4.8 beats/min with 10.6 and −8.2 beats/min 95% LOA in the normal weight group; 0.9±5.9 beats/min with 12.4 and −10.6 beats/min 95% LOA in the overweight group; and 0.7±6.5 beats/min with 13.5 and −12.1 beats/min 95% LOA in the obese group (Figure 5A). For HR, a bias of −2.0±12.9 beats/min with 23.3 and −27.3 beats/min 95% LOA was found in the underweight group; 0.8±6.5 beats/min with 13.6 and −11.9 beats/min 95% LOA in the normal weight group; −1.9±12.6 beats/min with 22.8 and −26.7 beats/min 95% LOA in the overweight group; and 8.2±19.2 beats/min with 46 and −29.5 beats/min 95% LOA in the obese group (Figure 6A). Measurements of RR in all BMI groups and HR in the normal weight group showed small mean bias levels between the radar and the ECG. Compared to HR, RR showed higher accuracy from all BMI groups. Next, we also analyzed the RR and HR data of all BMI groups defined by gender (Figures 5B, 5C and 6B, 6C). The measurements of RR and HR of all male BMI groups from the radar were more accurate.
Discussion
Conventional monitoring systems for assessing vital signs are electrocardiography (ECG), which requires physicians and medical staff to have direct contact with the patient and collect data using sensors attached to the body. However, with the COVID-19 pandemic, we are facing the most extreme stress and impact on our lives around the world and have also faced growing challenges in hospital healthcare. Image-based methods and radar-based methods are the main contactless health surveillance methods [19,20]. Contactless patient monitoring technologies can facilitate early detection of vital signs in patients [21], while reducing the risk of infection among physicians and medical staff during the COVID-19 pandemic.
In previous works, we introduced and validated a method for non-contact SIL radar using a single antenna-based system model in laboratory tests [8] and small-scale clinical studies [21]. We were able to monitor the vital signs of multiple people with multiple SIL radars in laboratory tests [7,8]. However, use of a single SIL radar for multiple people in the hospital needs to be investigated. According to our study examining the opportunities associated with contactless medical equipment during or after the COVID-19 pandemic, the contactless single SIL radar offer safer management in the hospital, but the accuracy of signal record between the SIL radar collection and the ECG needs to be improved. Our Bland-Altman analysis showed that SIL radar provided better signal accuracy for clinical monitoring than the contact-based ECG method of breath and heart rates across individual user or multiusers, genders, and BMI groups. We demonstrated that the signal accuracy for clinical monitoring could be measured accurately in the single person group with those measured using the conventional ECG. In addition, we showed that the measurements of vital signs of the male subgroup from radar were more accurate. In all BMI groups, the data of RR showed more accuracy than the HR. The main advantage of the SIL radar system is the ability to perform contactless and accurate monitoring of RR.
This clinical investigation has several limitations. First, not all measurements were accurate for respiratory rate and heartbeat rate detection in our SIL radar. In the case of respiratory rates in all BMI groups and heartbeat rates in the normal BMI group, there were small mean bias levels of discrepancy in the measurement results between the radar and the ECG, but there were inaccuracies between the radar and the ECG in the results of heartbeat rates in abnormal BMI group [22]. Compared to other radar systems, we were able to obtain more accurate measurements of respiratory rates with the SIL radar [23], but the inaccurate heartbeat rate results could not be used in overweight users with physical instability. Obesity and other metabolic syndromes associated with heartbeat status are affected by body composition, such as body fat [24,25]. Body fat with physical instability was correlated with heartbeat rates. Therefore, in this study, we identified the inaccuracy of heartbeat rate measurements in obese groups using SIL radar. Second, a challenge for contactless monitoring is health management of patients with chronic illnesses who require continuous medical care with SIL radar in the hospital. Our radar system for accurate validation of patients with chronic diseases needs to be improved. Third, this was a single-center study, and multi-center studies with larger samples are needed.
The Bland-Altman method is a statistical analysis used to compare accuracy between 2 methods (SIL radar and ECG in this study) using 95% limits of agreement (LOA) to assess agreement between 2 different methods [14]. Accuracy is associated with bias and the limits of agreement (LOA) [18]. Further investigation showed the correlation between 2 methods with Pearson correlation coefficient. However, the scatter plots revealed that the correlation between these methods lacked accuracy.
Conclusions
SIL radar can detect the respiratory and heartbeat beats of participants in the hospital with the most effective and accurate measurements. We assessed the SIL radar system in males and females and in various and BMI groups, showing accuracies in respiratory and heartbeat beats for single users and male users. The SIL radar presented could be a possible option for contactless monitoring of vital signs in hospitals. However, more testing is needed to examine these measurements accuracies and to validate their usefulness and limitations for other patient populations.
Figures
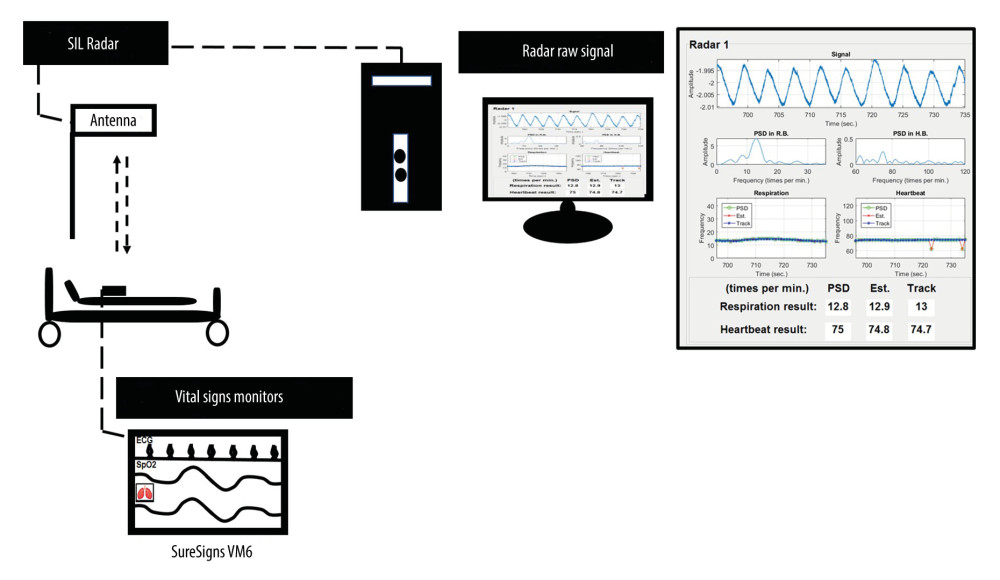
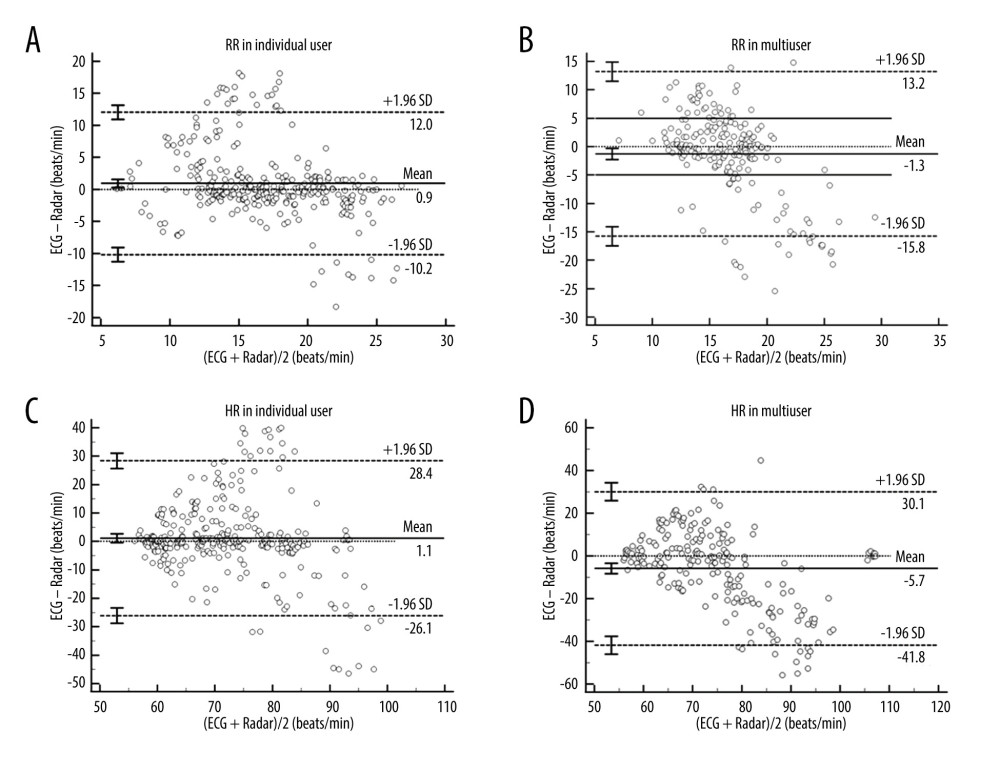
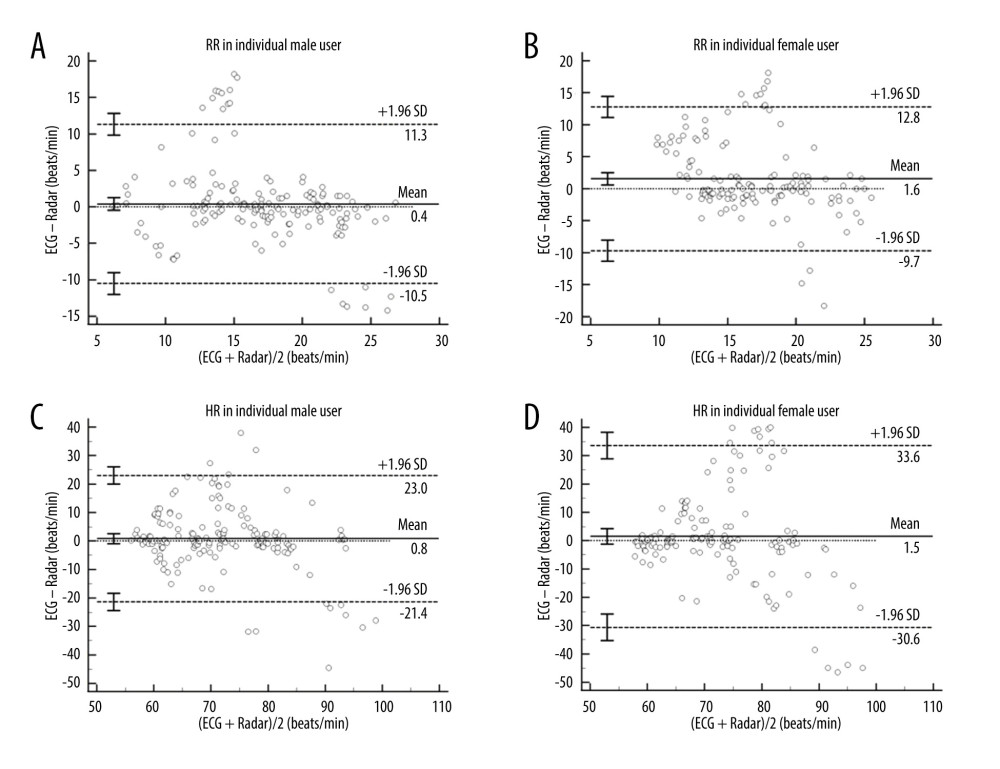
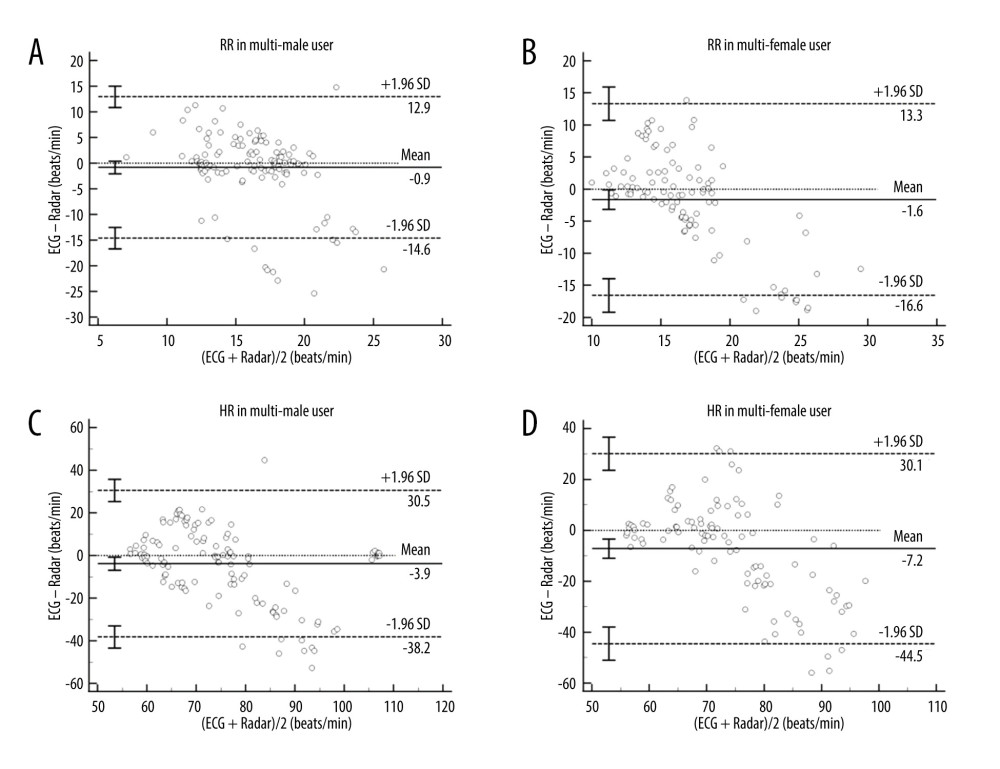
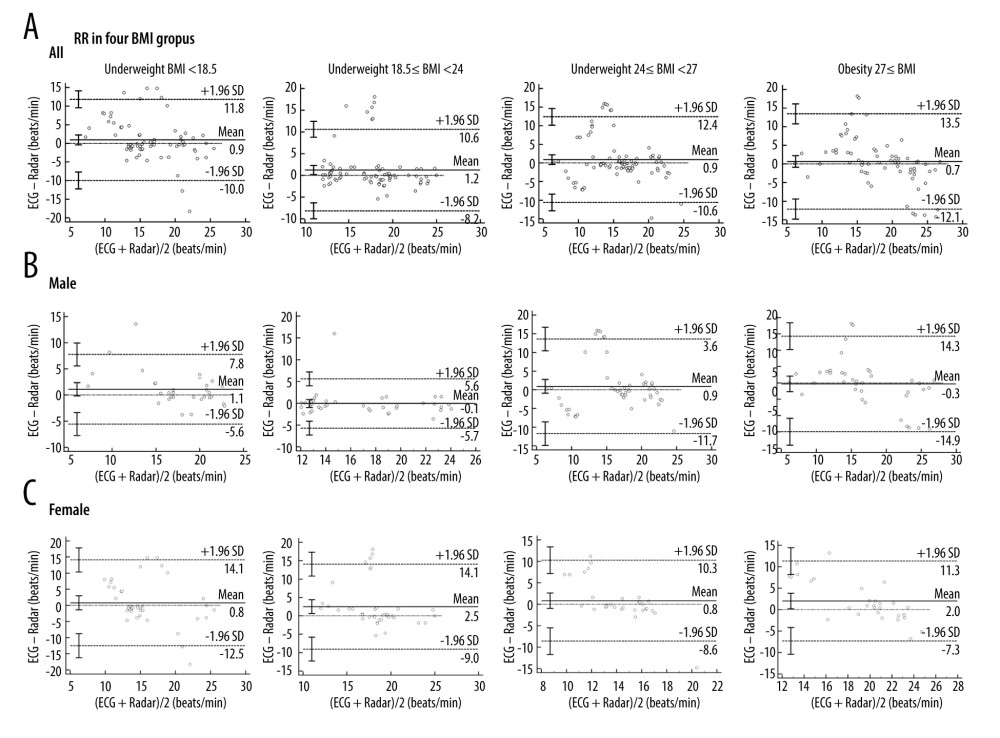
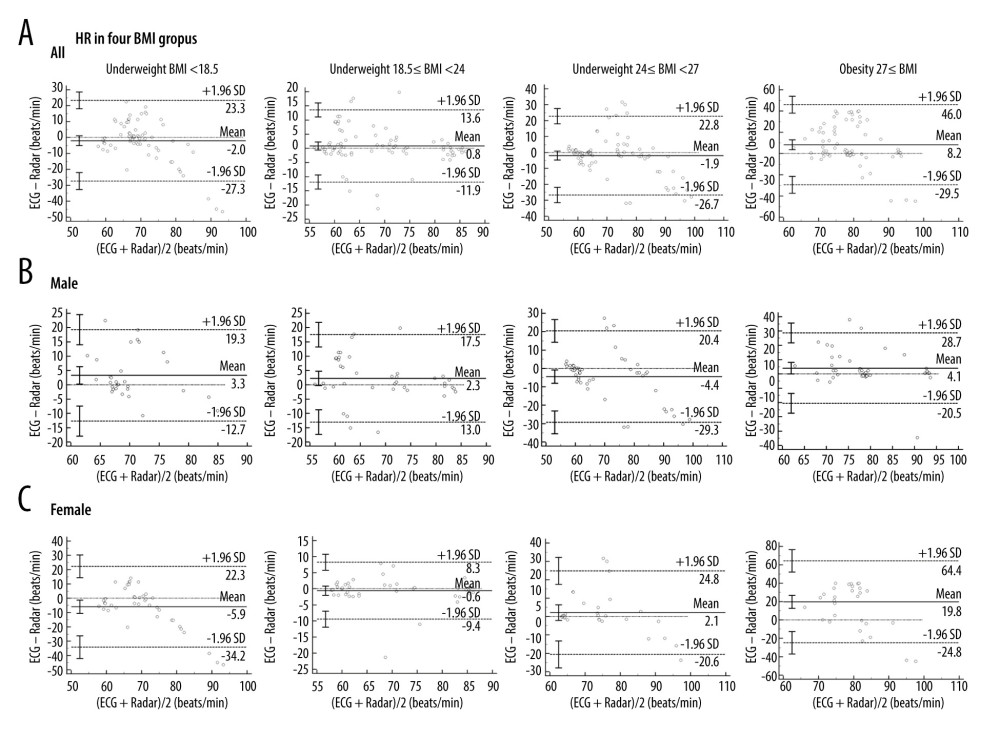
References
1. WHO Guidelines Approved by the Guidelines Review Committee Guideline: Assessing and Managing Children at Primary Health-Care Facilities to Prevent Overweight and Obesity in the Context of the Double Burden of Malnutrition: Updates for the Integrated Management of Childhood Illness (IMCI), 2017, Geneva, World Health Organization Available at: https://www.who.int/publications/i/item/9789241550123
2. Phua J, Weng L, Ling L, Intensive care management of coronavirus disease 2019 (COVID-19): Challenges and recommendations: Lancet Respir Med, 2020; 8(5); 506-17
3. Liu J, Teng G, Hong F, Human activity sensing with wireless signals: A survey: Sensors (Basel), 2020; 20(4); 1210
4. Massaroni C, Nicolò A, Sacchetti M, Schena E, Contactless methods for measuring respiratory rate: A review: IEEE Sensors Journal, 2021; 12821-39
5. Ni A, Azarang A, Kehtarnavaz N, A review of deep learning-based contactless heart rate measurement methods: Sensors (Basel), 2021; 21(11); 3719
6. Wang FK, Tang MC, Su SC, Horng TS, Wrist pulse rate monitor using self-injection-locked radar technology: Biosensors (Basel), 2016; 6(4); 54
7. Chian DM, Wen CK, Wang CJ, Vital signs identification system with Doppler radars and thermal camera: IEEE Trans Biomed Circuits Syst, 2022; 16(1); 153-67
8. Chian DM, Wen CK, Wang FK, Wong KK, Signal separation and tracking algorithm for multi-person vital signs by using Doppler radar: IEEE Trans Biomed Circuits Syst, 2020; 14(6); 1346-61
9. Shi K, Schellenberger S, Michler F, Automatic signal quality index determination of radar-recorded heart sound signals using ensemble classification: IEEE Trans Biomed Eng, 2020; 67(3); 773-85
10. Lee Y, Park JY, Choi YW, A novel non-contact heart rate monitor using impulse-radio ultra-wideband (IR-UWB) radar technology: Sci Rep, 2018; 8(1); 13053
11. Mercuri M, Lorato IR, Liu Y-H, Vital-sign monitoring and spatial tracking of multiple people using a contactless radar-based sensor: Nature Electronics, 2019; 252-62
12. Hamilton C, Lewis S, The importance of using the correct bounds on the Bland-Altman limits of agreement when multiple measurements are recorded per patient: J Clin Monit Comput, 2010; 24(3); 173-75
13. Zou GY, Confidence interval estimation for the Bland-Altman limits of agreement with multiple observations per individual: Stat Methods Med Res, 2013; 22(6); 630-42
14. Giavarina D, Understanding Bland Altman analysis: Biochem Med (Zagreb), 2015; 25(2); 141-51
15. Sedgwick P, Limits of agreement (Bland-Altman method): BMJ, 2013; 346; f1630
16. Zaki R, Bulgiba A, Ismail NA, Testing the agreement of medical instruments: Overestimation of bias in the Bland-Altman analysis: Prev Med, 2013; 57(Suppl); S80-82
17. Martin Bland J, Altman D, Statistical methods for assessing agreement between two methods of clinical measurement: Lancet, 1986; 1(8476); 307-10
18. Montenij LJ, Buhre WF, Jansen JR, Methodology of method comparison studies evaluating the validity of cardiac output monitors: A stepwise approach and checklist: Br J Anaesth, 2016; 116(6); 750-58
19. Schroder S, Loftfield N, Langmann B, Contactless operating table control based on 3D image processing; 2014; 388-92
20. Iwata Y, Thanh HT, Sun G, Ishibashi K, High accuracy heartbeat detection from CW-Doppler radar using singular value decomposition and matched filter: Sensors (Basel), 2021; 21(11); 3588
21. Tsai CY, Chang NC, Fang HC, A novel non-contact self-injection-locked radar for vital sign sensing and body movement monitoring in COVID-19 isolation ward: J Med Syst, 2020; 44(10); 177
22. Shyu KK, Chiu LJ, Lee PL, Detection of breathing and heart rates in UWB radar sensor data using FVPIEF-based two-layer EEMD: IEEE Sensors Journal, 2019; 774-84
23. Kuutti J, Paukkunen M, Aalto M, Evaluation of a Doppler radar sensor system for vital signs detection and activity monitoring in a radio-frequency shielded room: Measurement, 2015; 135-42
24. Ahmed S, Lee Y, Lim YH, Noncontact assessment for fatigue based on heart rate variability using IR-UWB radar: Sci Rep, 2022; 12(1); 14211
25. Triggiani AI, Valenzano A, Trimigno V, Heart rate variability reduction is related to a high amount of visceral adiposity in healthy young women: PLoS One, 2019; 25(14); e0223058
Figures
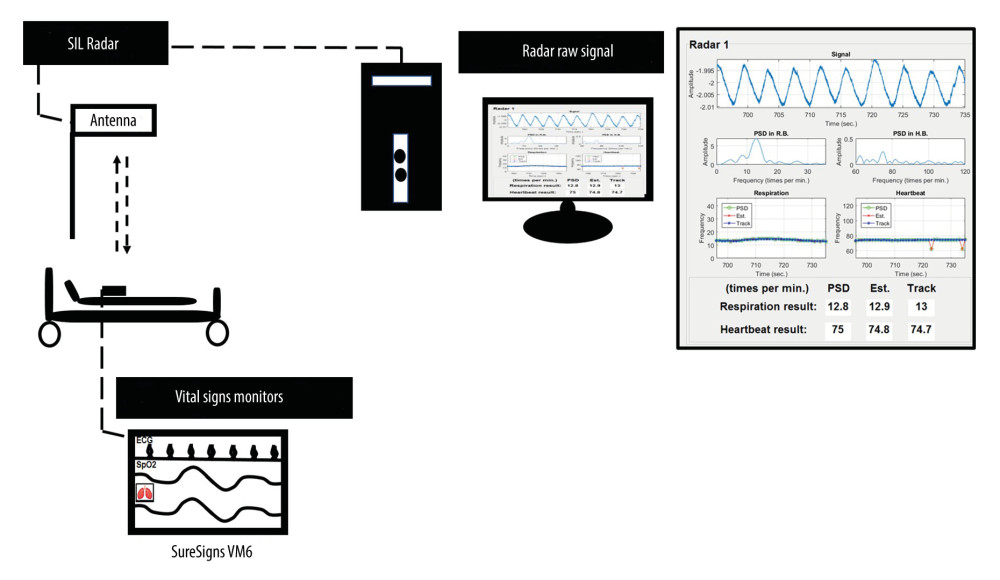
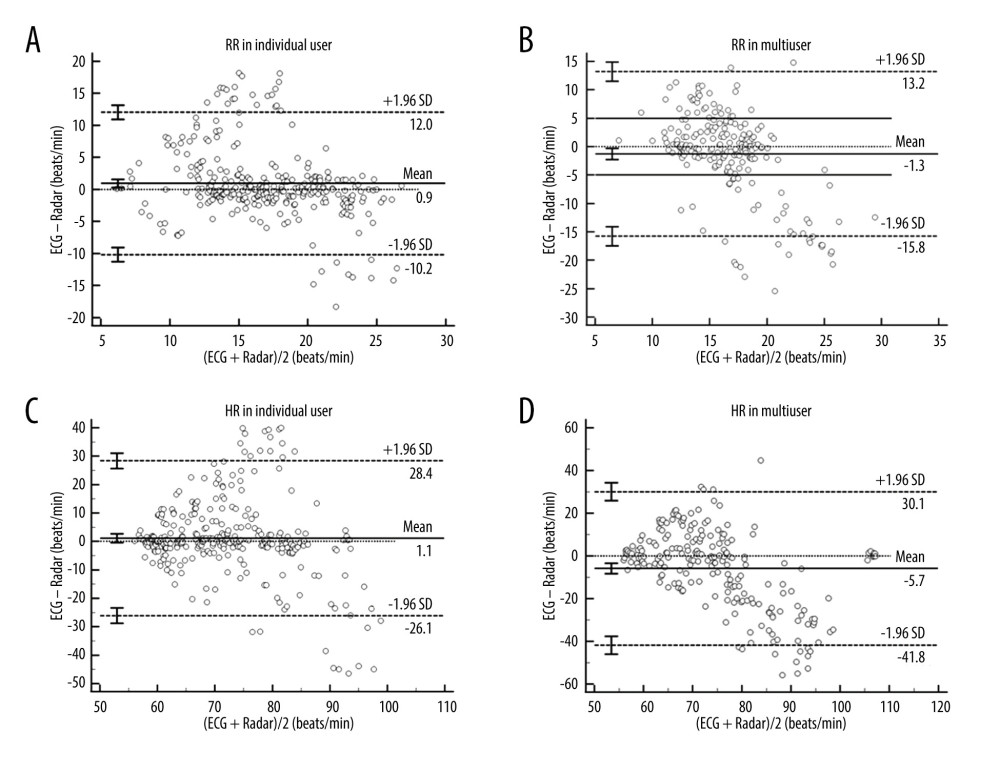
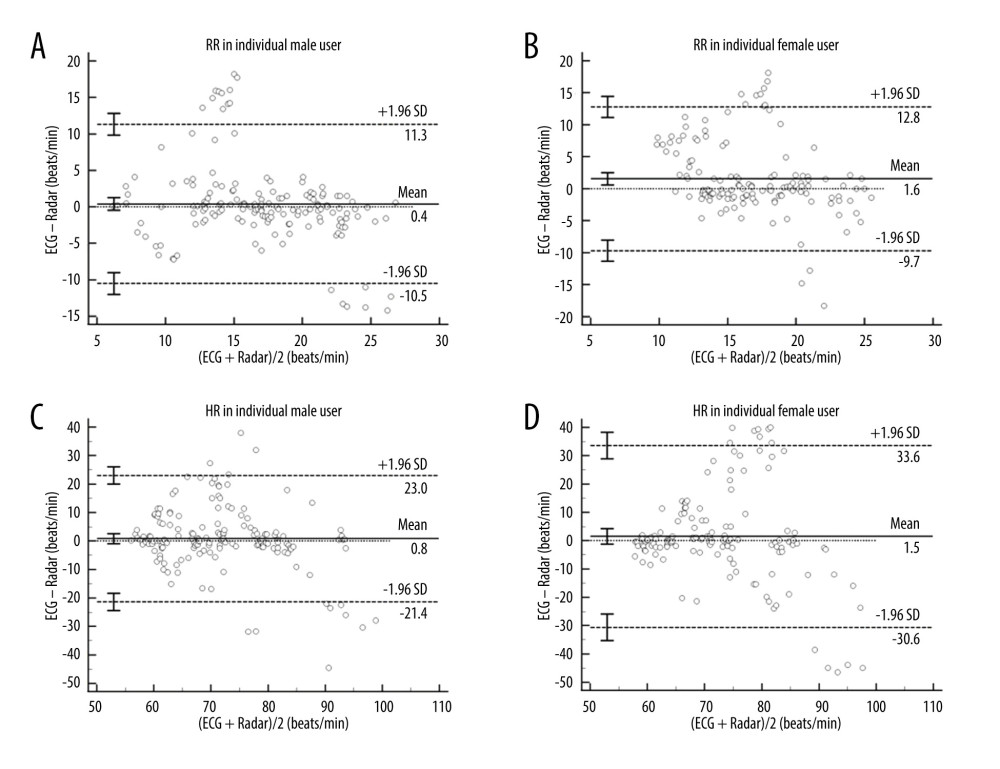
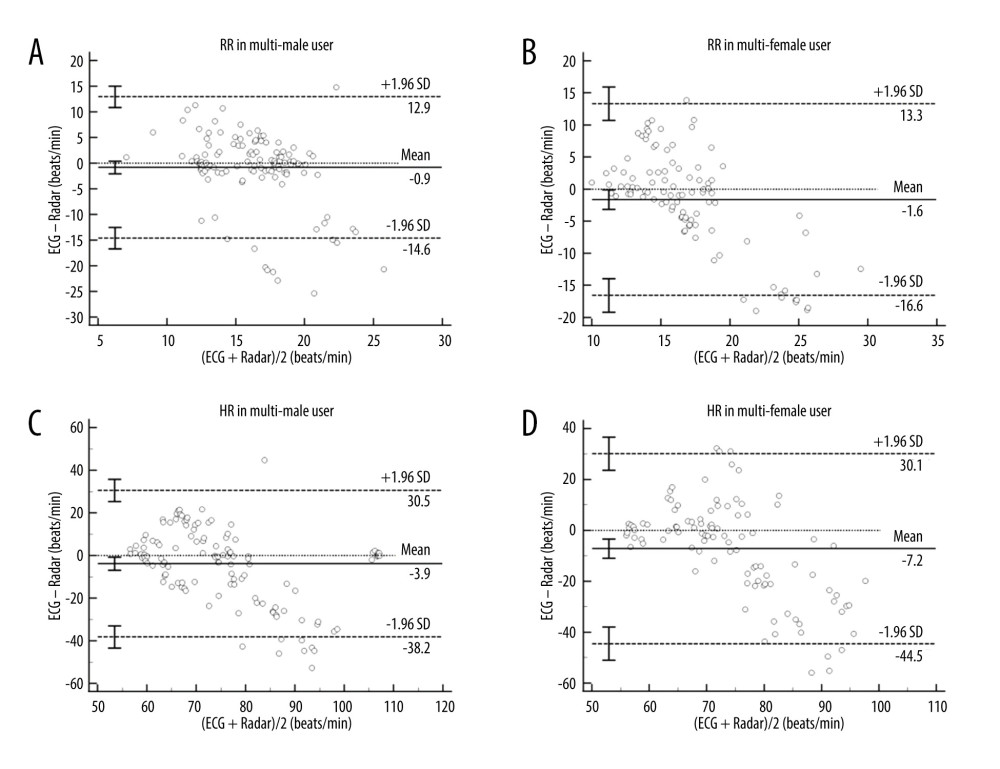
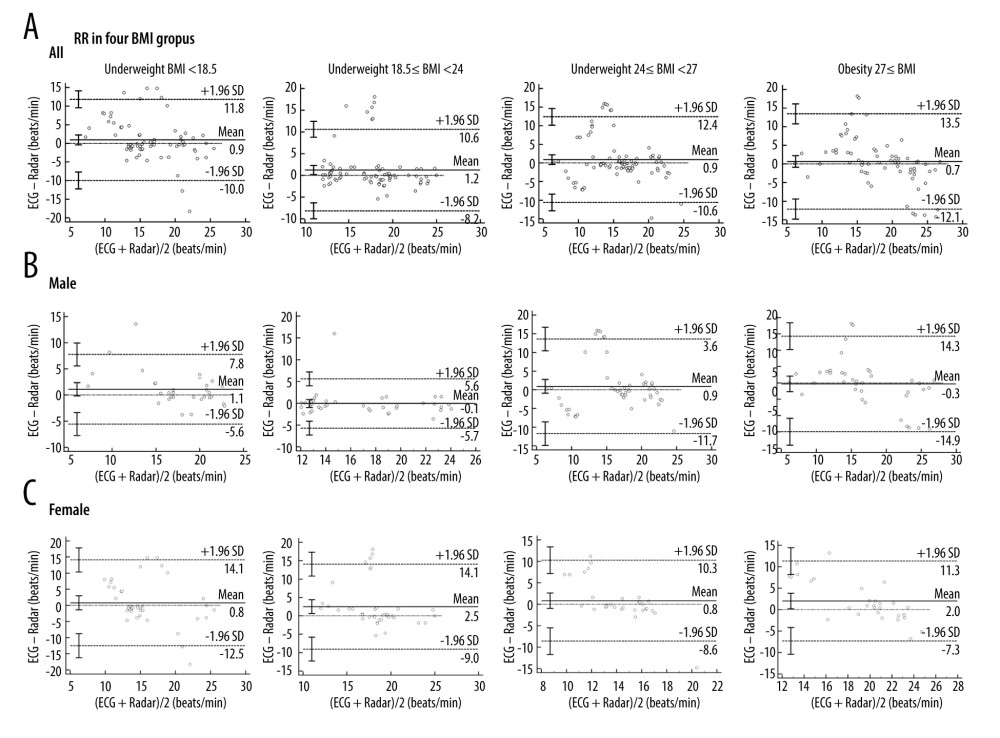
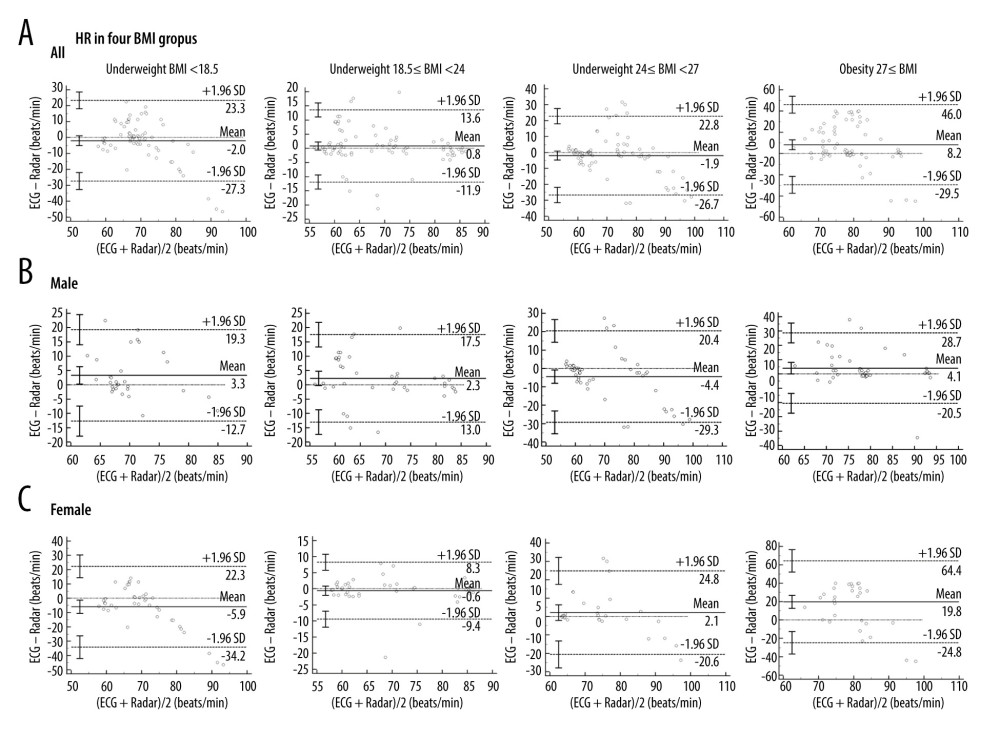
In Press
07 Mar 2024 : Clinical Research
Knowledge of and Attitudes Toward Clinical Trials: A Questionnaire-Based Study of 179 Male Third- and Fourt...Med Sci Monit In Press; DOI: 10.12659/MSM.943468
08 Mar 2024 : Animal Research
Modification of Experimental Model of Necrotizing Enterocolitis (NEC) in Rat Pups by Single Exposure to Hyp...Med Sci Monit In Press; DOI: 10.12659/MSM.943443
18 Apr 2024 : Clinical Research
Comparative Analysis of Open and Closed Sphincterotomy for the Treatment of Chronic Anal Fissure: Safety an...Med Sci Monit In Press; DOI: 10.12659/MSM.944127
08 Mar 2024 : Laboratory Research
Evaluation of Retentive Strength of 50 Endodontically-Treated Single-Rooted Mandibular Second Premolars Res...Med Sci Monit In Press; DOI: 10.12659/MSM.944110
Most Viewed Current Articles
17 Jan 2024 : Review article
Vaccination Guidelines for Pregnant Women: Addressing COVID-19 and the Omicron VariantDOI :10.12659/MSM.942799
Med Sci Monit 2024; 30:e942799
14 Dec 2022 : Clinical Research
Prevalence and Variability of Allergen-Specific Immunoglobulin E in Patients with Elevated Tryptase LevelsDOI :10.12659/MSM.937990
Med Sci Monit 2022; 28:e937990
16 May 2023 : Clinical Research
Electrophysiological Testing for an Auditory Processing Disorder and Reading Performance in 54 School Stude...DOI :10.12659/MSM.940387
Med Sci Monit 2023; 29:e940387
01 Jan 2022 : Editorial
Editorial: Current Status of Oral Antiviral Drug Treatments for SARS-CoV-2 Infection in Non-Hospitalized Pa...DOI :10.12659/MSM.935952
Med Sci Monit 2022; 28:e935952