31 December 2023: Clinical Research
Generation of a Predictive Clinical Model for Isolated Distal Deep Vein Thrombosis (ICMVT) Detection
Yan Xu1ABCDE, Mingmin Xu1CD, Xiaofang Zheng1CD, Fengxia Jin1BCD, Bin Meng1ABCDFG*DOI: 10.12659/MSM.942840
Med Sci Monit 2023; 29:e942840
Abstract
BACKGROUND: Isolated distal deep vein thrombosis (ICMVT) increases the risk of pulmonary embolism. Although predictive models are available, their utility in predicting the risk is unknown. To develop a clinical prediction model for isolated distal calf muscle venous thrombosis, data from 462 patients were used to assess the independent risk variables for ICMVT.
MATERIAL AND METHODS: The area under curve (AUC) for Model A and Model B were calculated and other risk factors were based on age, pitting edema in the symptomatic leg, calf swelling with least 3 cm larger than the asymptomatic leg, recent bed rest for 3 days or more in the past 4 weeks, requiring general or major surgery with regional anesthesia, sex, and local tenderness distributed along the deep venous system as independent predictors of calf muscle venous thrombosis. Model A includes the risk variables for C-reactive protein and D-dimer.
RESULTS: The area under ROC curve for Model A training set was 0.924 (95% CI: 0.895-0.952), the area under ROC curve for Model B training set was 0.887 (95% CI: 0.852-0.922), and the AUC difference between the 2 models was statistically significant (P<0.001); the area under ROC curve for Model A obtained in the validation set was 0.902 (95% CI: 0.844-0.961), the area under ROC curve for Model B was 0.842 (95% CI: 0. 0.773-0.910), and the difference between the 2 models was statistically significant (P=0.012).
CONCLUSIONS: Predictive Model A better predicts isolated calf muscle venous thrombosis and is able to help clinicians rapidly and early diagnose ICMVT, displaying higher utility for missed diagnosis prevention and disease therapy.
Keywords: nomograms, Risk Factors, Venous Thrombosis
Background
Isolated distal deep vein thrombosis (ICMVT) commonly occurs in muscular veins, and similar studies have revealed that more than 75% of deep vein thromboses occur in muscular calf veins (MCVT) [1]. MCVT development is considered to be a peripheral type of deep vein thrombosis, often located in the venous plexus of the gastrocnemius and soleus muscles [2,3], accounting for 5.6–31.3% of lower-extremity DVTs [4,5]. International guidelines and relevant research recommend anticoagulation for patients with ICMVT (eg, hospitalization, history of VTE, and cancer). Continuous venous ultrasonography monitoring without commencing anticoagulation or short-term anticoagulation may be recommended in low-risk individuals [6–10]. In asymptomatic individuals with IDDVT, continuous DUS monitoring and maintenance of mechanical prophylaxis may be an effective approach to preventing proximal thrombus extension and recurrence [11–15].
Although research on thrombosis has focused on prevention and treatment and has produced great progress [16–22], its early prediction has emerged as a significant research focus. According to the American Society of Hematology’s 2018 guidelines for the diagnosis of venous thromboembolism and recommendations from the Society of Radiologists at the Ultrasound Consensus Conference, some vascular ultrasounds may not systematically evaluate the calf and gastrocnemius veins and may miss nearly half of all calf DVTs [3,23]. Given the high rate of misdiagnosis of calf muscle venous thrombosis (ICMVT) [24–26], our model can help minimize missed diagnoses while also providing clinical benefit for patient prognosis. In this study, a prediction model was established. Although several ICMVT risk factors have been found, few combination criteria have been identified to construct a clinical prediction model for ICMVT.
This study used ICMVT patients’ initial admission information, as well as a series of clinical data and laboratory tests, to develop a concise prediction model that predicted ICMVT in the form of a dynamic nomogram, which is a graphical computer tool used in clinical predictive modeling [27–29]. In this project, we aimed to develop a user-friendly predictive model for patients with ICMVT. The implementation of this model is expected to have significant implications in clinical settings, as it can potentially mitigate the likelihood of misdiagnosis, minimize the need for excessive examinations, and alleviate the burden on patients. In the event that therapy is deemed necessary, the attainment of a precise diagnosis becomes imperative. To mitigate patient costs, it is advisable to minimize the frequency of ultrasound examinations in cases where subsequent evaluations are required. Consequently, developing a prediction model for the diagnosis of ICMVT is important.
Material and Methods
PATIENTS’ DATA:
Patients hospitalized in our hospital between January 2016 and December 2020 were retrospectively analyzed. A total of 462 patients were divided into 2 groups, the thrombus group, and the non-thrombus group, based on the results of vascular color Doppler ultrasound. The thrombosis group (N=208, 45%) consisted of 96 males (46.2%) and 112 females (53.8%), with a mean age of 72.4±13.84 years. The non-thrombus group consisted of 151 males (59.4%) and 103 females (40.6%), with a mean age of 60.83±17.09 years (Figure 1). The inclusion criteria were: (1) age ≥16 years, and (2) complete ultrasound and clinical data.
The exclusion criteria were: (1) unclear image and imprecise diagnosis, (2) insufficient clinical data (incomplete laboratory tests), (3) a history of thrombosis or the use of antithrombotic drugs (eg, low-molecular-weight Heparin, aspirin), and (4) combined with other parts of the thrombus. Figure 1 depicts a detailed flowchart of the screening procedure. According to the requirements for establishing a clinical prediction model, the sample size should be at least 10 times the number of variables. Our study had 18 variables; thus, the sample size should be at least 180, and accordingly, our sample size is sufficient.
DIAGNOSIS OF LOWER-EXTREMITY DEEP VEIN THROMBOSIS:
With a probe frequency of 5–12 MHz, a Philips EPIQ5 ultrasonic diagnostic device was employed. A full duplex ultrasound routine from the thigh to the ankle, using compression and color Doppler ultrasonography at chosen areas, is recommended by the Society of Ultrasound Radiologists [30]. Color Doppler ultrasonography diagnostic criteria for lower-extremity calf muscle venous thrombosis mainly include: (1) An abnormal echo in the official cavity, (2) The vein under the ultrasonography probe cannot be closed by pressing, (3) No visible blood flow signal in the venous thrombus section, and (4) The blood vessel diameter and blood flow of the afflicted limb are diminished.
DATA COLLECTION AND ACQUISITION:
Three components were used for data collection through literature research: (1) patient demographics, such as sex, age, etc.; (2) medical history, such as active cancer (ongoing or within the past 6 months of treatment or palliative care), paralysis, or recent lower-extremity cast, recent bed rest for 3 days or more, or major surgery requiring general or regional anesthesia within the past 4 weeks, localized tenderness along the deep venous system, swelling of the entire leg, swelling of the calf at least 3 cm larger than the asymptomatic leg (measured at 10 cm below the tibial tubercle), previously recorded DVT, pitting edema in the symptomatic leg, etc.; and (3) After admission, laboratory test results with a white blood cell count, neutrophil count, lymphocyte count, platelet count, PLT volume, C-reactive protein, fibrinogen (FIB), D-dimer, and neutrophil percentage. Data were collected on the first day of admission or the earliest day after admission. The data for this study were collected and retrieved by 2 attending physicians with 10 years of experience, according to the clinical information system. This study was approved by the Medical Ethics Committee of Zhejiang Invalids Hospital and Zhejiang Rongjun Hospital (approval number: 2021 Lun Shen Yan No. 15), and the requirement for informed consent was waived.
MODEL BUILDING:
Taking into consideration the convenience of clinical application, we employed multivariate logistic regression and least absolute shrinkage and selection operator (LASSO) regression to screen all variables. LASSO assists in simplifying the model by increasing the penalty coefficient (λ) to compress the estimated value of each variable [31]. Following that, the best model is selected by comparing NRI and IDI. The AIC is utilized as the model screening termination criteria since the smaller the AIC, the less the prediction error.
Multivariate logistic regression analysis and LASSO regression were used to identify the independent risk variables for isolated calf muscle venous thrombosis using a database of hospitalized patients from January 2016 to December 2020. Models A and B were developed to predict isolated distal calf muscle venous thrombosis based on the presence or absence of laboratory tests. Model A includes laboratory tests such as the risk variables for C-reactive protein and D-dimer. To analyze and evaluate the final clinical impact, the accuracy and stability of the 2 models were evaluated using the operating characteristic curve (ROC), calibration curve, integrated discrimination index (IDI), net reclassification index (NRI), the prediction of isolated calf using decision curve (DCA), and the construction dynamic nomogram of muscle venous thrombosis.
STATISTICAL ANALYSIS:
All statistical analyses were performed using IBM SPSS Statistics for Windows version 25.0 (IBM Corporation, Armonk, NY, USA). Computer programs were used to randomly divide the research population into 3: 1 training and validation samples, and categorical variables were reported as percentages (%) indicated and analyzed using the chi-square test as appropriate. After performing univariate logistic regression analysis, significant variables with
Results
DEMOGRAPHIC AND CLINICAL CHARACTERISTICS:
This study comprised a total of 462 patients, ranging in age from 16 to 97 years (66.02±16.72). The research population was divided into 2 groups – the training group and the verification group – with 328 in the training group and 134 in the verification group. The verification group’s average age was 66.13±16.71 years old, with 62 (46.3%) being female patients. The indicators in both samples were consistent (Table 1). Except for the calf swelling being at least 3 cm greater than the asymptomatic leg (P>0.05), no significant differences were identified between the 2 groups.
RISK FACTORS FOR DEVELOPMENT OF ICMVT:
Univariate logistic regression analysis distributed sex, age, paralysis, paralysis or recent lower-extremity cast fixation, recent bed rest for 3 days or more, major surgery requiring general or regional anesthesia in the past 4 weeks, local tenderness distributed along the deep venous system, whole-leg swelling, calf swelling at least 3 cm larger than the asymptomatic leg, previously documented DVT, pitting edema in symptomatic leg, lymphocyte count, PLT volume, CRP, FIB, D-dimer, and neutrophils. The percentage differences were statistically significant (
Multivariate logistic regression analysis showed age [OR: 1.98; 95% CI: 1.21–3.25; P=0.007], CRP (OR: 3.25; 95% CI: 1.08–9.79; P=0.037), symptomatic leg symptoms pitting edema (OR: 3.52; 95% CI: 1.67–7.4; P=0.001), D-dimer (OR: 2.42; 95% CI: 1.22–4.82; P=0.011), lower-leg swelling was at least 3 cm larger than that of the asymptomatic leg (OR: 2.68; 95% CI: 1.37–5.25; P=0.004), sex (OR: 2.24; 95% CI: 1.44–3.51; P<0.001), bedridden for 3 or more days recently or 4 major surgeries requiring general or regional anesthesia within a week (OR: 11.35; 95% CI: 3.88–33.15; P=0.006), local tenderness distributed along the deep venous system (OR: 13.11; 95% CI: 3.83–44.88; P<0.001) was an independent predictor of calf muscle venous thrombosis (Table 2). LASSO regression screening variables (Figure 2) selected 7 clinical features (recent bed rest for 3 days or more, major surgery requiring general or regional anesthesia within the past 4 weeks, localized tenderness along the deep venous system, whole-leg swelling, pitting edema in symptomatic legs, age, D-dimer, CRP) to construct the optimal model when the NRI and IDI of the 2 models constructed by multi-factor logistic regression and LASSO regression screening variables were compared, NRI (categorical variable) was 0.065 (95% CI: 0.017–0.113; p=0.008), NRI (continuous variable) was 0.363 (95% CI: 0.149–0.578; P=0.001), IDI 0.017 (95% CI: −6e-04-0.034; P=0.050). Ultimately, recent immobilization greater than 3 days or major surgery within the past 4 weeks, localized tenderness of deep venous system, calf swelling greater than 3 cm compared with asymptomatic side, unilateral pitting edema, age, D-dimer, and CRP were taken into consideration to construct a nomogram for predicting isolated calf muscle venous thrombosis.
ESTABLISHMENT AND VERIFICATION OF ICMVT NOMOGRAM:
Eventually, this study established 8 factors to construct the prediction Model A (the minimum AIC value was 246.60). In comparison, Model B has no laboratory test findings. To evaluate the recognition performance of the models, we calculated the AUC of Model A and Model B. The area under the ROC curve for Model A training set was 0.924 (95% CI: 0.895–0.952), the diagnostic threshold was 0.50, the sensitivity was 0.901, and the specificity was 0.844; the area under the ROC curve for Model B training set was 0.887 (95% CI: 0.852–0.922), the diagnostic threshold was 0.447, the sensitivity was 0.836, and the specificity was 0.792 (Figure 3); the area under the ROC curve for Model A in the validation set was 0.902 (95% CI: 0.844–0.961), and the diagnostic threshold was 0.488, the sensitivity was 0.951, the specificity was 0.778, the area under the ROC curve for Model B was 0.842 (95% CI: 0.0.773–0.910), the diagnostic threshold was 0.432, the sensitivity was 0.780, and the specificity was 0.722 (Figure 3).
The AUC difference between the 2 models in the training set was statistically significant (Z=3.352, P=0.001), as was the difference between the 2 models in the validation set (Z=2.5224, P=0.012). The NRI classification variable was 0.1108 (95% CI: 0.038–0.184; P=0.003), the NRI continuous variable was 0.8902 (95% CI: 0.696–1.084, P<0.001), IDI 0.0964 (95% CI: 0.065–0.127, P<0.001), NRI greater than 0, showed positive improvement, indicating that the predictive ability of the model has improved after adding the laboratory indicators D-dimer and CRP, and the proportion of correct classification had increased by 11.1%. This work utilized R to generate a calibration curve for the H-L (Hosmer-Lemeshow) test on the training and verification sets throughout the model verification procedure (Figure 4). The curve indicates that the prediction model fits the actual scenario.
CLINICAL APPLICATION OF NOMOGRAM IN PREDICTING ICMVT:
DCA illustrates the clinical usefulness of Model A and Model B in the training and validation sets (Figure 5). The DCA curve demonstrated that if the diagnosis with a predicted probability of 50% is selected as treatment for ICMVT patients, 29 patients benefited from Model A and 19 patients benefited from Model B, out of 100 patients in total. Model A has a higher line, indicating that predicting Model A leads to a higher net gain than predicting Model B. In addition, using R to construct a dynamic nomogram has a high prediction capacity for the occurrence of ICMVT. It employed Model A to present the corresponding assignments and 95% reliability intervals obtained by 8 individuals at various risk variables. The results were helpful in improving the practicability of the nomogram, and directly observing the risk factor score of ICMVT as well as the probability of ICMVT (Table 3).
Discussion
Relevant studies have shown that using the Wells score and D-dimer detection is the first step in the diagnosis of DVT, but the efficiency of this approach, known as ICMVT, is limited [32,33]. In this study, some of the predictors in the Wells score were selected, and laboratory tests were used to construct a predictive model. It was found that adding D-dimer and CRP to construct a predictive model for ICMVT has a greater clinical value. Considering that the research population is mostly ICMVT patients, different research populations may result in differences in research outcomes. Another study found that age, sex, paralysis of the lower extremities, and malignant tumors are all independent risk factors for early MCVT [34,35], which is consistent with the independent risk factors established by the results of our study: age, sex, recent bed rest for 3 days or more, major surgery requiring general or regional anesthesia in the past 4 weeks. Multiple studies have shown that tumors can significantly increase the risk of VTE [36–39]. However, this study found that tumors are not a risk factor for ICMVT. Compared to proximal deep vein thrombosis (PDVT) patients and patients with pulmonary embolism (PE) in the lower extremities, ICMVT patients are more likely to undergo surgery or experience lower-extremity trauma, while PDVT Or PE patients are more likely to suffer from invasive cancer [38]. Here, the results are consistent with previous findings [38,40,41].
Galanaud et al [42] investigated the risk factors that influence recurrence of distal deep vein thrombosis, and concluded that age above 50 years was a risk factor. In this study, the age of 50 years was used as the cut-off point to divide the research population into 3 groups: younger than 50 years old, 50–60 years old, and older than 60 years old. Age was found to be an independent risk factor. Related studies have shown that the frequency of distal DVT in women is higher than that in men [25,43,44], which is consistent with the findings of this research that sex is an independent risk factor for ICMVT.
This study found that the preoperative VTE incidence rate of femoral neck fracture patients was 18.9%, with a DVT incidence rate of 18.9%, and among the DVT cases, 77.2% were ICMVT [45]. D-dimer is an independent risk factor for ICMVT [46], with a reference value for detecting early judgment of lower-extremity DVT. The findings of dynamic detection and evaluation of D-dimer level help diagnose and monitor DVT in its early stages [47]. Many studies have focused on the use of early detection methods, especially for blood D-dimer levels, to predict the occurrence of DVT. However, the degree of trauma, the degree of inflammatory response, age, and other variables all contribute to a rise in D-dimer levels [48]. A study showed that preoperative D-dimer levels >1.0 mg/L were associated with a 3.5 increased risk of preoperative DVT in patients with severe fractures [49,50]. It should be emphasized, however, that the concentration of D-dimer in cancer patients is generally higher, particularly in patients with invasive cancer [26,28,51,52]. Age-adjusted D-dimer values have been widely used to predict deep vein thrombosis [53–56]. Following the multivariate logistic regression, it was discovered in this study that D-dimer was an independent risk factor for ICMVT (OR: 2.42; 95% CI: 1.22–4.82;
This study examined the clinical data of a single center over the last 5 years to establish a clinical prediction model and predict the risk of ICMVT in these patients. The predictors used in this model are recurrent and easily identifiable in clinical practice, which can help clinicians monitor the risk of ICMVT on a regular basis and reduce the expense of routine imaging scans. The calibration curve depicts the consistency of the predicted and the observed risk of ICMVT, assuring the repeatability and reliability of the test. In DCA, the net benefit is calculated by subtracting the predicted benefits from the projected hazards associated with each prediction model [57–59]. In the validation set, DCA revealed that Model A outperformed Model B in predicting the risk of ICMVT patients. The dynamic nomogram can directly observe the risk factor score of ICMVT and the probability of ICMVT in the prediction model. Therefore, an accurate evaluation of the risk of ICMVT will help guide the use of antithrombotic medicines in clinical practice.
There are various drawbacks to this study. First, the final nomogram was established based on retrospective analysis of a single-center database. As a result, a large-scale multicenter study is required to verify the final nomogram’s generalizability in additional centers. Second, because retrospective studies are prone to selection bias, prospective external validation is necessary. New methodologies for future prediction models are required to help clinical decision-making and lead future research.
Conclusions
By comparing the clinical feature model to the combined model through laboratory data, we discovered that the combined model had the highest diagnostic performance. As a result, we were able to successfully design a dynamic nomogram based on clinical and laboratory data that accurately predicted ICMVT. The nomogram is an easy-to-use tool with high accuracy and capacity to quickly diagnose the ICMVT unit’s early stages and help clinicians make decisions and implement effective preventative interventions based on the risks of specific patients.
Figures
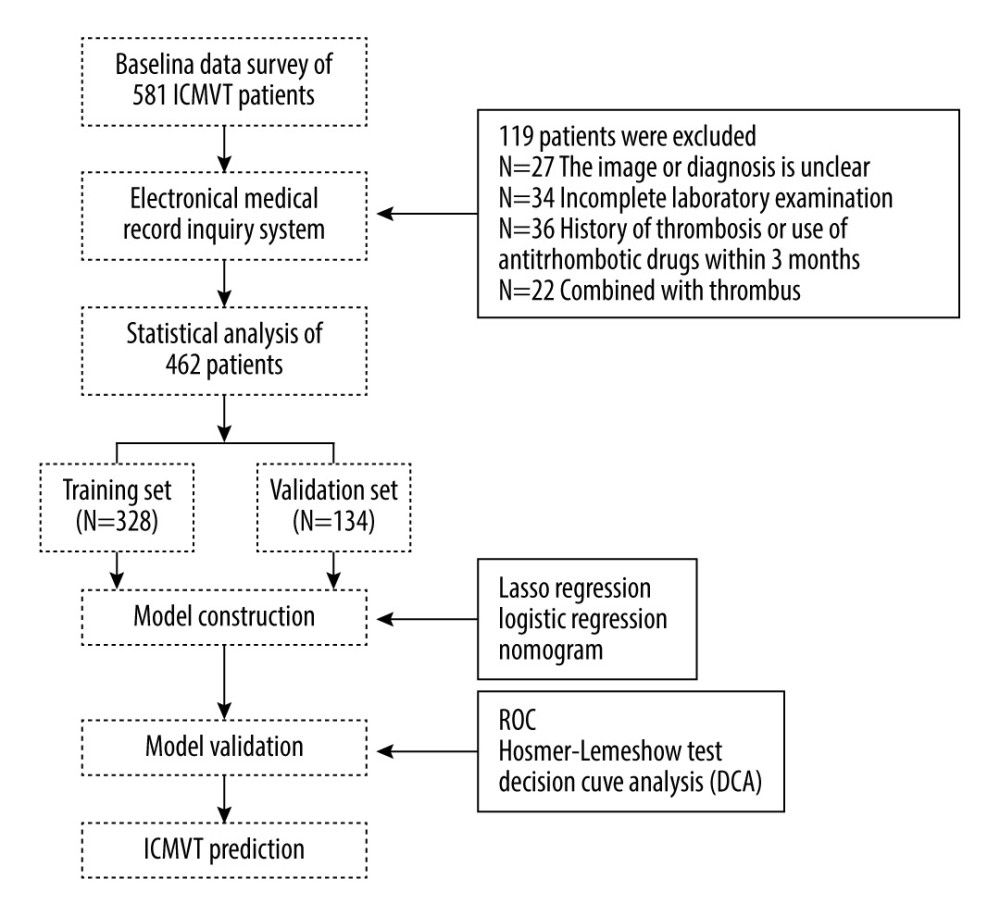
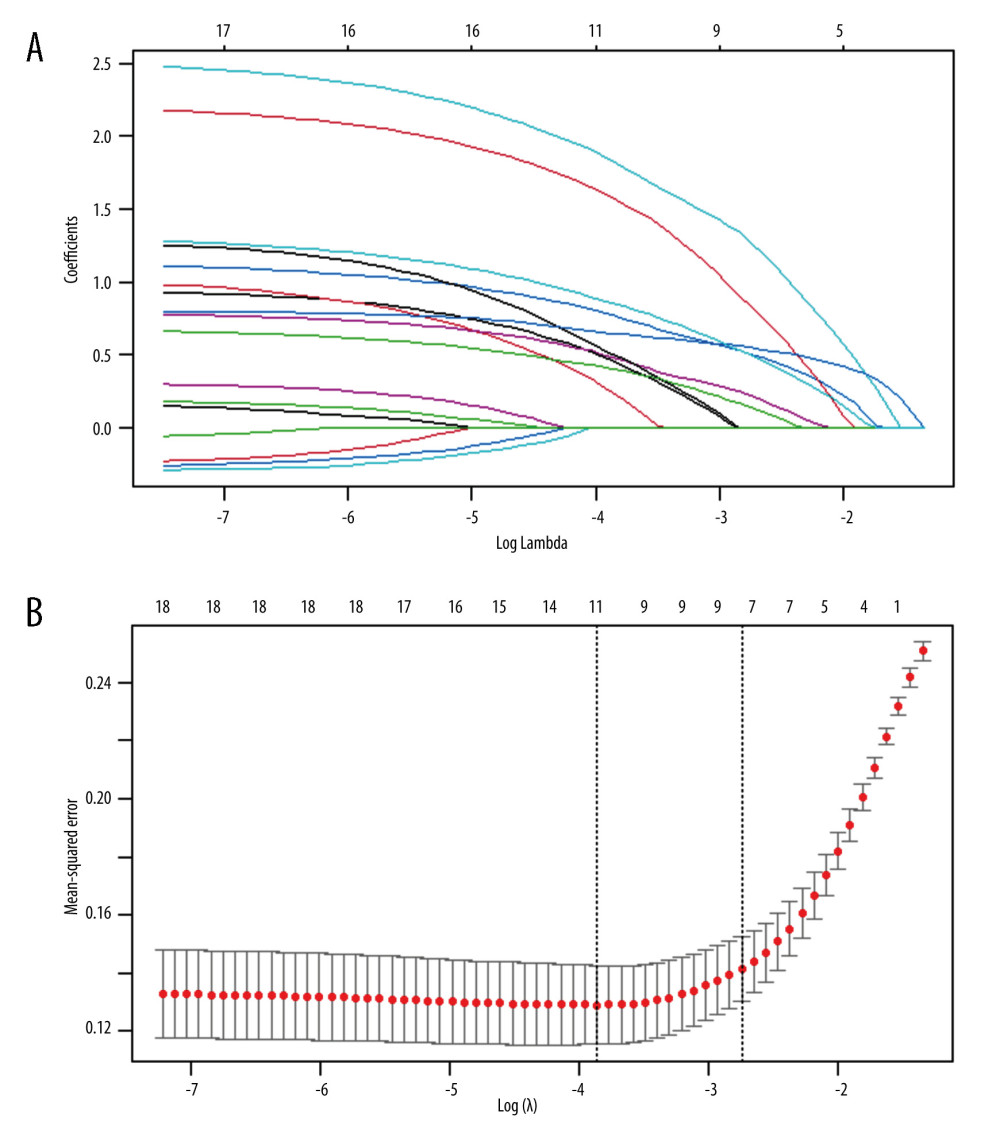
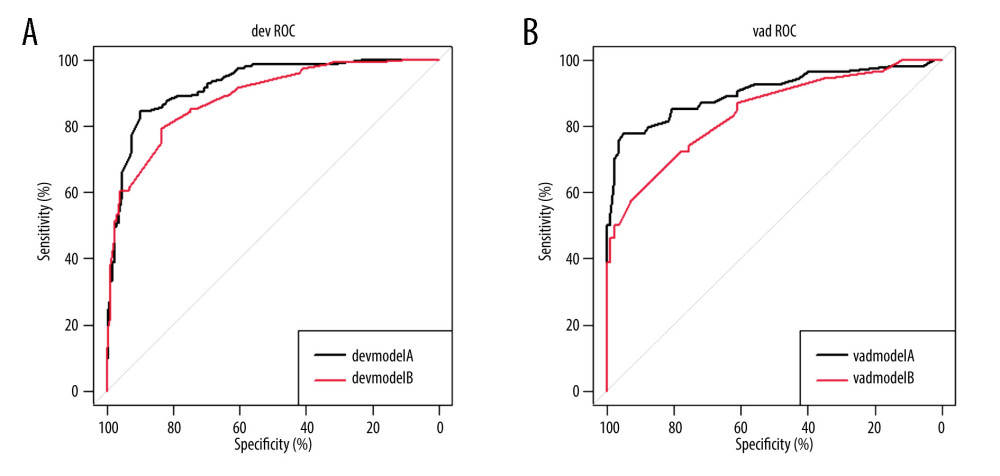
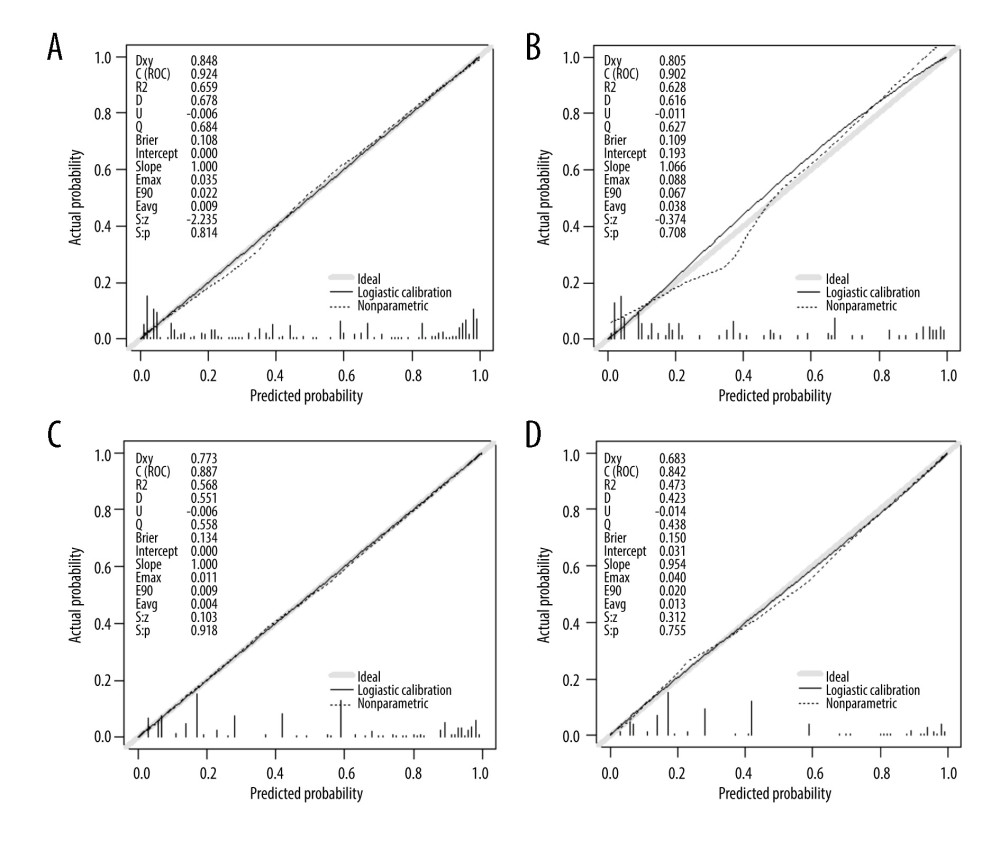
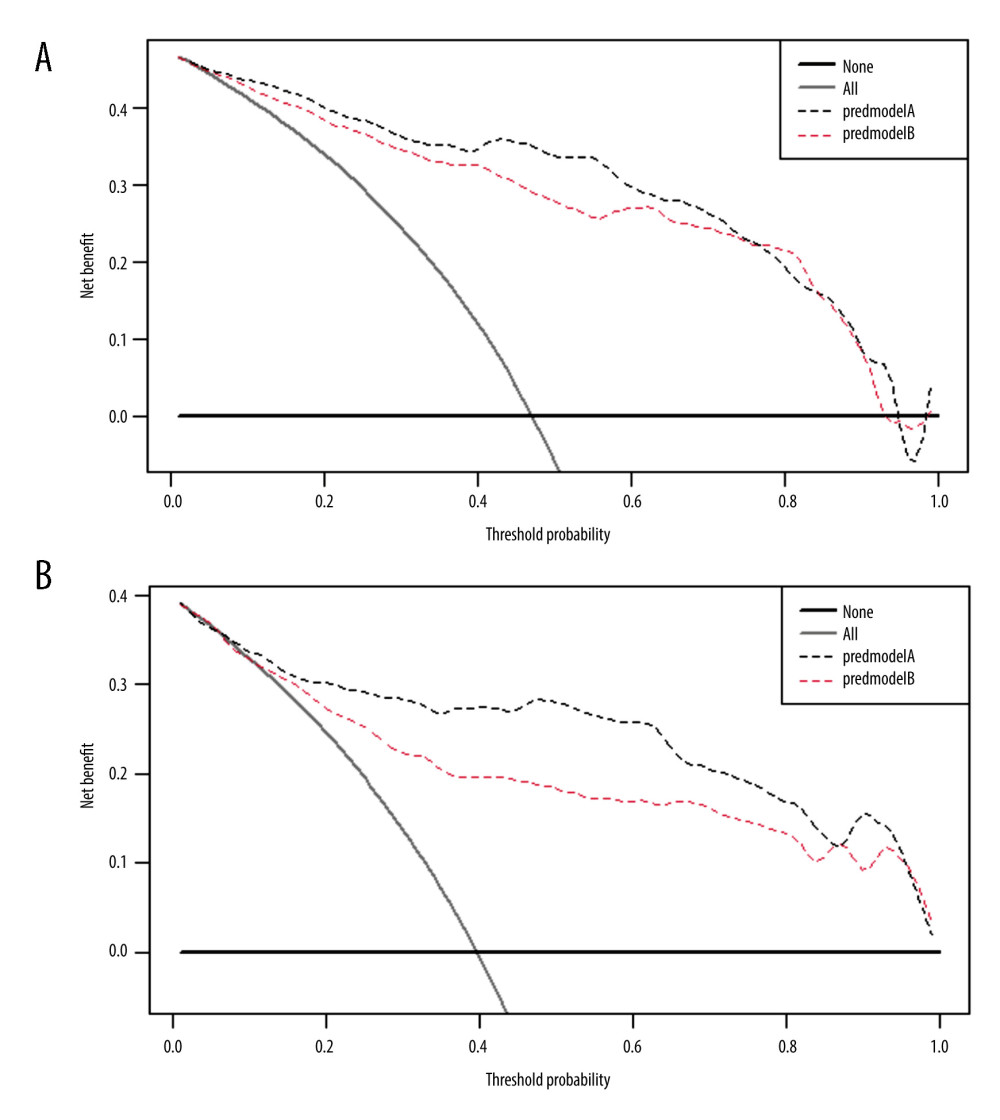
References
1. Wang B, Wang Q, Ji Y, The clinical outcomes of different doses of rivaroxaban in patients with isolated distal deep vein thrombosis: J Vasc Surg Venous Lymphat Disord, 2023 Online ahead of print
2. Zhang P, Bian Y, Xu F, The incidence and characteristics of venous thromboembolism in neurocritical care patients: A prospective observational study: Clin Appl Thromb Hemost, 2020; 26; 1076029620907954
3. Kuczmik W, Wysokinski WE, Hesley GK, Calf vein thrombosis comparison of outcomes for axial and muscular venous thrombosis: Thromb Haemost, 2021; 121(2); 216-23
4. Henry JC, Satiani B, Calf muscle venous thrombosis: A review of the clinical implications and therapy: Vasc Endovascular Surg, 2014; 48(5–6); 396-401
5. Potere N, Ageno W, How to treat isolated distal deep vein thrombosis: Pol Arch Intern Med, 2023; 133(7–8); 16543
6. Mazzolai L, Aboyans V, Ageno W, Diagnosis and management of acute deep vein thrombosis: A joint consensus document from the European Society of Cardiology working groups of aorta and peripheral vascular diseases and pulmonary circulation and right ventricular function: Eur Heart J, 2018; 39(47); 4208-18
7. Kearon C, Akl EA, Ornelas J, Antithrombotic therapy for VTE disease: CHEST Guideline and Expert Panel Report: Chest, 2016; 149(2); 315-52
8. Chopard R, Albertsen IE, Piazza G, Diagnosis and treatment of lower extremity venous thromboembolism: A review: JAMA, 2020; 324(17); 1765-76
9. Merriman E, Chunilal S, Brighton T, Two weeks of low molecular weight heparin for isolated symptomatic distal vein thrombosis (TWISTER study): Thromb Res, 2021; 207; 33-39
10. Galanaud JP, Trujillo-Santos J, Bikdeli B, Clinical presentation and outcomes of patients with cancer-associated isolated distal deep vein thrombosis: J Clin Oncol, 2023 Online ahead of print
11. Kim MH, Jun KW, Hwang JK, Incidence and outcome of isolated distal deep vein thrombosis in kidney transplant recipients: Ann Surg Treat Res, 2020; 98(6); 324-31
12. Galanaud JP, Sevestre MA, Pernod G, Isolated distal deep vein thrombosis: What have we learnt from the OPTIMEV study?: J Med Vasc, 2023; 48(1); 3-10
13. Brown C, Brandt W, Wang TF, Incidence of recurrent venous thromboembolism and bleeding complications in patients with cancer and isolated distal deep vein thrombosis: Thromb Res, 2023; 228; 81-84
14. Luo X, Zhang L, Hou C, Hospitalized patients with isolated distal deep vein thrombosis: Anticoagulation therapy or not?: Thromb J, 2022; 20(1); 52
15. Day T, Tran H, Chunilal S, Isolated distal DVT in trauma: A study of the management of isolated distal deep vein thrombosis acquired as an inpatient in trauma patients: Injury, 2022; 53(7); 2562-66
16. Chang JC, Pathogenesis of two faces of DVT: New identity of venous thromboembolism as combined micro-macrothrombosis via unifying mechanism based on “two-path unifying theory” of hemostasis and “two-activation theory of the endothelium”: Life (Basel), 2022; 12(2); 220
17. Zhou M, Zhang W, Zhang Y, Therapeutic or prophylactic anticoagulation in acute isolated distal deep vein thrombosis: Protocol for a prospective, multicentre, single-blind, randomised controlled trial (TOP-IDDVT): BMJ Open, 2022; 12(2); e056826
18. Schellong S, Ageno W, Casella IB, Profile of patients with isolated distal deep vein thrombosis versus proximal deep vein thrombosis or pulmonary embolism: RE-COVERY DVT/PE Study: Semin Thromb Hemost, 2022; 48(4); 446-58
19. Vlashi R, Zhang X, Wu M, Chen G, Wnt signaling: Essential roles in osteoblast differentiation, bone metabolism and therapeutic implications for bone and skeletal disorders: Genes Dis, 2023; 10(4); 1291-317
20. Juma SN, Liao J, Huang Y, Osteoarthritis versus psoriasis arthritis: Physiopathology, cellular signaling, and therapeutic strategies: Genes & Diseases, 2023 in press
21. Huang Y, Liao J, Vlashi R, Chen G, Focal adhesion kinase (FAK): Its structure, characteristics, and signaling in skeletal system: Cell Signal, 2023; 110852
22. Hu S, Chen S, Zeng H, Ap-2beta regulates cranial osteogenic potential via the activation of Wnt/beta-catenin signaling pathway: Dev Biol, 2023; 501; 81-91
23. Lim W, Le Gal G, Bates SM, American Society of Hematology 2018 guidelines for management of venous thromboembolism: Diagnosis of venous thromboembolism: Blood Adv, 2018; 2(22); 3226-56
24. Makedonov I, Galanaud JP, Kahn SR, Significance and management of isolated distal deep vein thrombosis: Curr Opin Hematol, 2021; 28(5); 331-38
25. Li Y, Ding J, Shen L, Risk factors and anticoagulation therapy in patients with isolated distal deep vein thrombosis in the early post-operative period after thoracic surgery: Front Surg, 2021; 8; 671165
26. Galanaud JP, Trujillo-Santos J, Bikdeli B, Management of isolated distal deep-vein thrombosis with direct oral anticoagulants in the RIETE registry: J Thromb Thrombolysis, 2021; 52(2); 532-41
27. Park SY, Nomogram: An analogue tool to deliver digital knowledge: J Thorac Cardiovasc Surg, 2018; 155(4); 1793
28. Poudel SK, Park DY, Jia X, Clinical outcomes of isolated distal deep vein thrombosis versus proximal venous thromboembolism in cancer patients: The Cleveland Clinic experience: J Thromb Haemost, 2020; 18(3); 651-59
29. Shimabukuro N, Mo M, Hashiyama N, Clinical course of asymptomatic isolated distal deep vein thrombosis of the leg: A single-institution study: Ann Vasc Dis, 2019; 12(4); 487-92
30. Needleman L, Cronan JJ, Lilly MP, Ultrasound for lower extremity deep venous thrombosis: multidisciplinary recommendations from the Society of Radiologists in Ultrasound Consensus Conference: Circulation, 2018; 137(14); 1505-15
31. Wu L, Chang W, Song Y, Wang L, Predicting treatment failure risk in a Chinese Drug-Resistant Tuberculosis with surgical therapy: Development and assessment of a new predictive Nomogram: Int J Infect Dis, 2020; 96; 88-93
32. Sartori M, Cosmi B, Legnani C, The Wells rule and D-dimer for the diagnosis of isolated distal deep vein thrombosis: J Thromb Haemost, 2012; 10(11); 2264-69
33. Galanaud JP, Quenet S, Rivron-Guillot K, Comparison of the clinical history of symptomatic isolated distal deep-vein thrombosis vs. proximal deep vein thrombosis in 11 086 patients: J Thromb Haemost, 2009; 7(12); 2028-34
34. Cheng HR, Huang GQ, Wu ZQ, Individualized predictions of early isolated distal deep vein thrombosis in patients with acute ischemic stroke: A retrospective study: BMC Geriatr, 2021; 21(1); 140
35. Li AY, Woulfe T, Rolfe-Vyson V, Management and outcomes of axial isolated distal deep vein thrombosis at North Shore Hospital, New Zealand: A retrospective audit: Intern Med J, 2015; 45(2); 177-82
36. Shi J, Ye J, Zhuang X, Application value of Caprini risk assessment model and elevated tumor-specific D-dimer level in predicting postoperative venous thromboembolism for patients undergoing surgery of gynecologic malignancies: J Obstet Gynaecol Res, 2019; 45(3); 657-64
37. Tikkinen KAO, Craigie S, Agarwal A, Procedure-specific risks of thrombosis and bleeding in urological cancer surgery: Systematic review and meta-analysis: Eur Urol, 2018; 73(2); 242-51
38. Schellong SM, Goldhaber SZ, Weitz JI, Isolated distal deep vein thrombosis: Perspectives from the GARFIELD-VTE Registry: Thromb Haemost, 2019; 119(10); 1675-85
39. Donadini MP, Dentali F, Pegoraro S, Long-term recurrence of venous thromboembolism after short-term treatment of symptomatic isolated distal deep vein thrombosis: A cohort study: Vasc Med, 2017; 22(6); 518-24
40. Lim MS, Ariyarajah A, Oldmeadow C, A systematic review and meta-analysis comparing anticoagulation versus no anticoagulation and shorter versus longer duration of anticoagulation for treatment of isolated distal deep vein thrombosis: Semin Thromb Hemost, 2017; 43(8); 836-48
41. Ho P, Lim HY, Chua CC, Retrospective review on isolated distal deep vein thrombosis (IDDVT) – a benign entity or not?: Thromb Res, 2016; 142; 11-16
42. Galanaud JP, Sevestre MA, Genty C, Incidence and predictors of venous thromboembolism recurrence after a first isolated distal deep vein thrombosis: J Thromb Haemost, 2014; 12(4); 436-43
43. Trinchero A, Scheres LJJ, Prochaska JH, Sex-specific differences in the distal versus proximal presenting location of acute deep vein thrombosis: Thromb Res, 2018; 172; 74-79
44. Asonitis K, Charvalos E, Lambert C, Hermans C, Experience of a 40-day (6 week) LMWH treatment for isolated distal deep vein thrombosis: J Thromb Thrombolysis, 2020; 50(4); 837-43
45. Xia ZN, Xiao K, Zhu W, Risk assessment and management of preoperative venous thromboembolism following femoral neck fracture: J Orthop Surg Res, 2018; 13(1); 291
46. Chen C, Liu Y, Wu H, The outcome of isolated calf muscle vein thrombosis after open reduction and internal fixation for closed intra-articular distal femur fractures: A prospective cohort study: BMC Musculoskelet Disord, 2021; 22(1); 397
47. Li R, Jiang J, Song Y, Prognostic nomogram for predicting lower extremity deep venous thrombosis in Neurointensive Care Unit Patients: A Prospective observational study: Front Neurol, 2021; 12; 761029
48. Wang P, Kandemir U, Zhang B, Incidence and risk factors of deep vein thrombosis in patients with pelvic and acetabular fractures: Clin Appl Thromb Hemost, 2019; 25; 1076029619845066
49. Zhang W, Huai Y, Wang W, A Retrospective cohort study on the risk factors of deep vein thrombosis (DVT) for patients with traumatic fracture at Honghui Hospital: BMJ Open, 2019; 9(3); e024247
50. Juma SN, Gong X, Hu S, Shark new antigen receptor (IgNAR): Structure, characteristics and potential biomedical applications: Cells, 2021; 10(5); 1140
51. Liu B, Xie J, Sun X, Development and validation of a new clinical prediction model of catheter-related thrombosis based on vascular ultrasound diagnosis in cancer patients: Front Cardiovasc Med, 2020; 7; 571227
52. Asonitis K, Charvalos E, Lambert C, Hermans C, Experience of a 40-day (6 week) LMWH treatment for isolated distal deep vein thrombosis: J Thromb Thrombolysis, 2020; 50(4); 837-43
53. Broen K, Scholtes B, Vossen R, Predicting the need for further thrombosis diagnostics in suspected DVT is increased by using age adjusted D-dimer values: Thromb Res, 2016; 145; 107-8
54. Chen G, Xu H, Yao Y, BMP signaling in the development and regeneration of cranium bones and maintenance of calvarial stem cells: Front Cell Dev Biol, 2020; 8; 135
55. Chen G, Yao Y, Xu G, Zhang X, Regional difference in microRNA regulation in the skull vault: Dev Dyn, 2019; 248(10); 1009-19
56. Hu B, Wu T, Zhao Y, Physiological signatures of dual embryonic origins in mouse skull vault: Cell Physiol Biochem, 2017; 43(6); 2525-34
57. Vickers AJ, Elkin EB, Decision curve analysis: A novel method for evaluating prediction models: Med Decis Making, 2006; 26(6); 565-74
58. Fitzgerald M, Saville BR, Lewis RJ, Decision curve analysis: JAMA, 2015; 313(4); 409-10
59. Sartori M, Gabrielli F, Favaretto E, Proximal and isolated distal deep vein thrombosis and Wells score accuracy in hospitalized patients: Intern Emerg Med, 2019; 14(6); 941-47
Figures
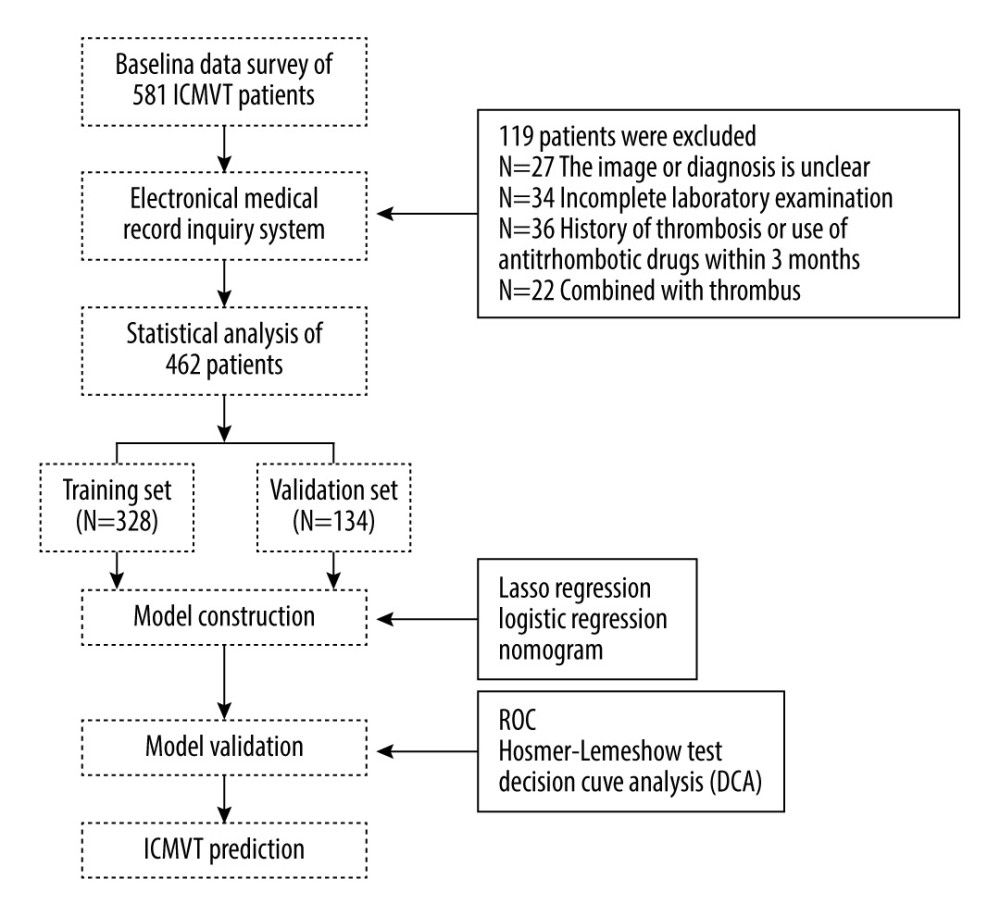
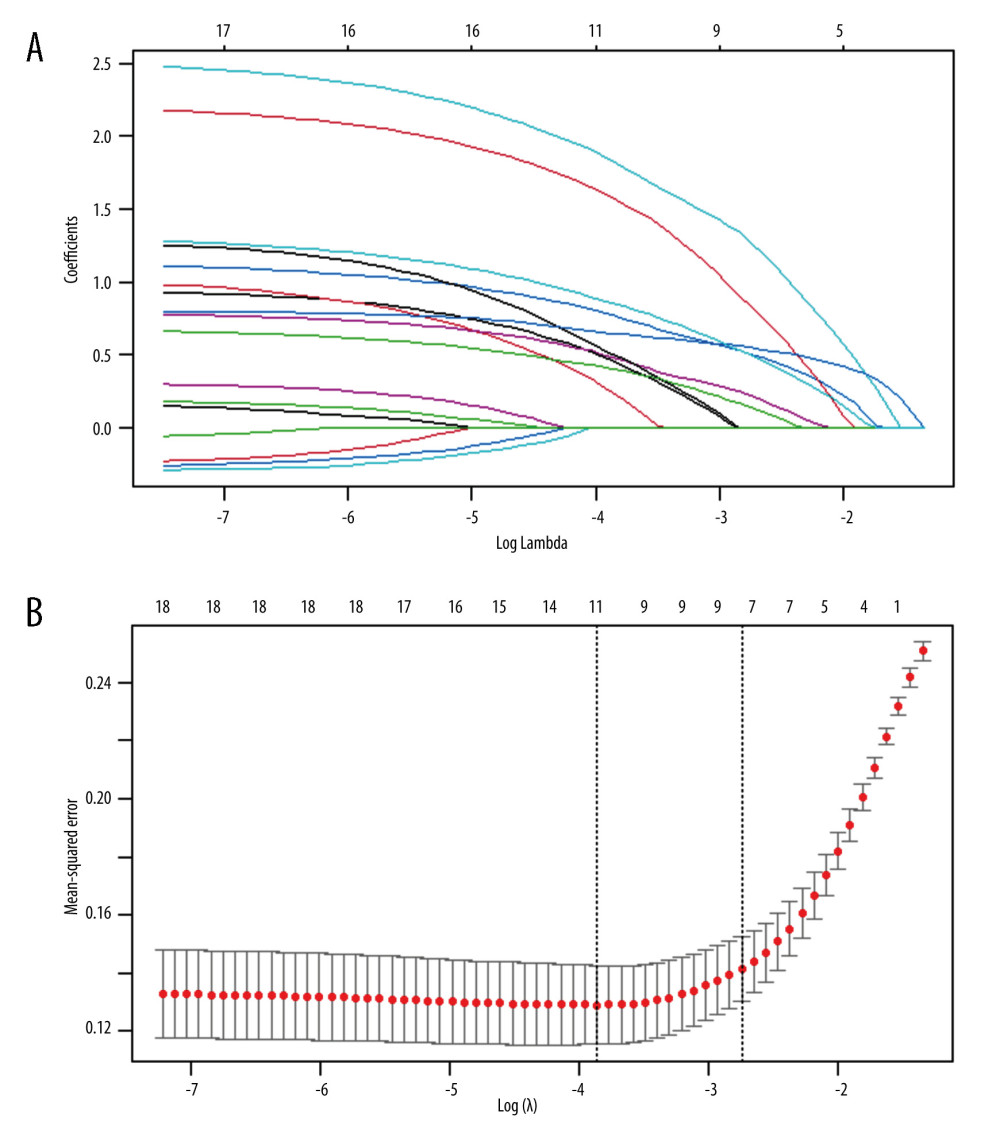
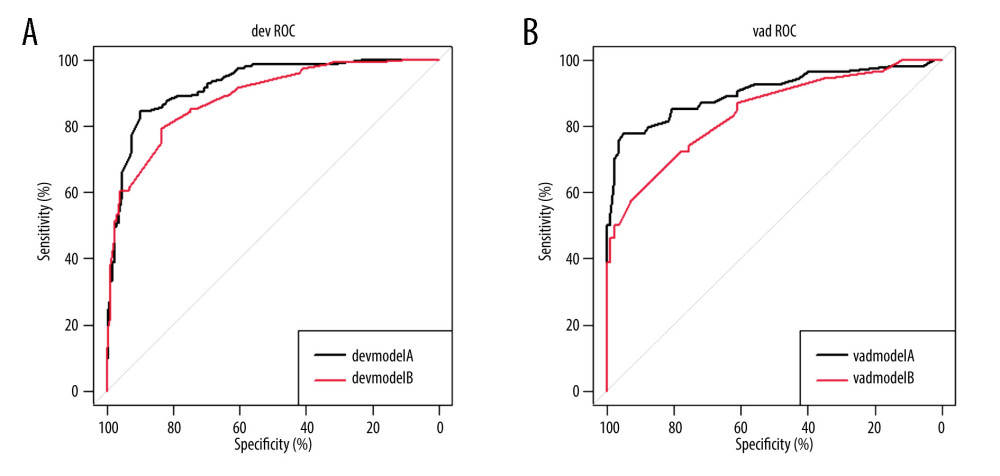
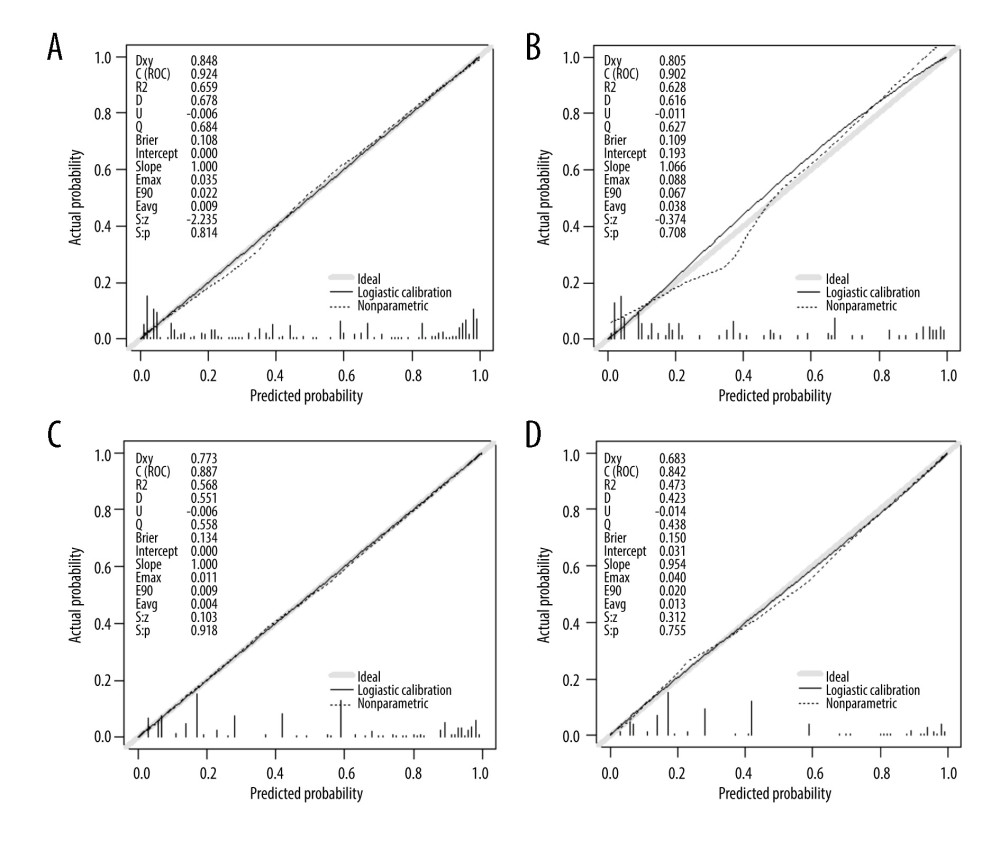
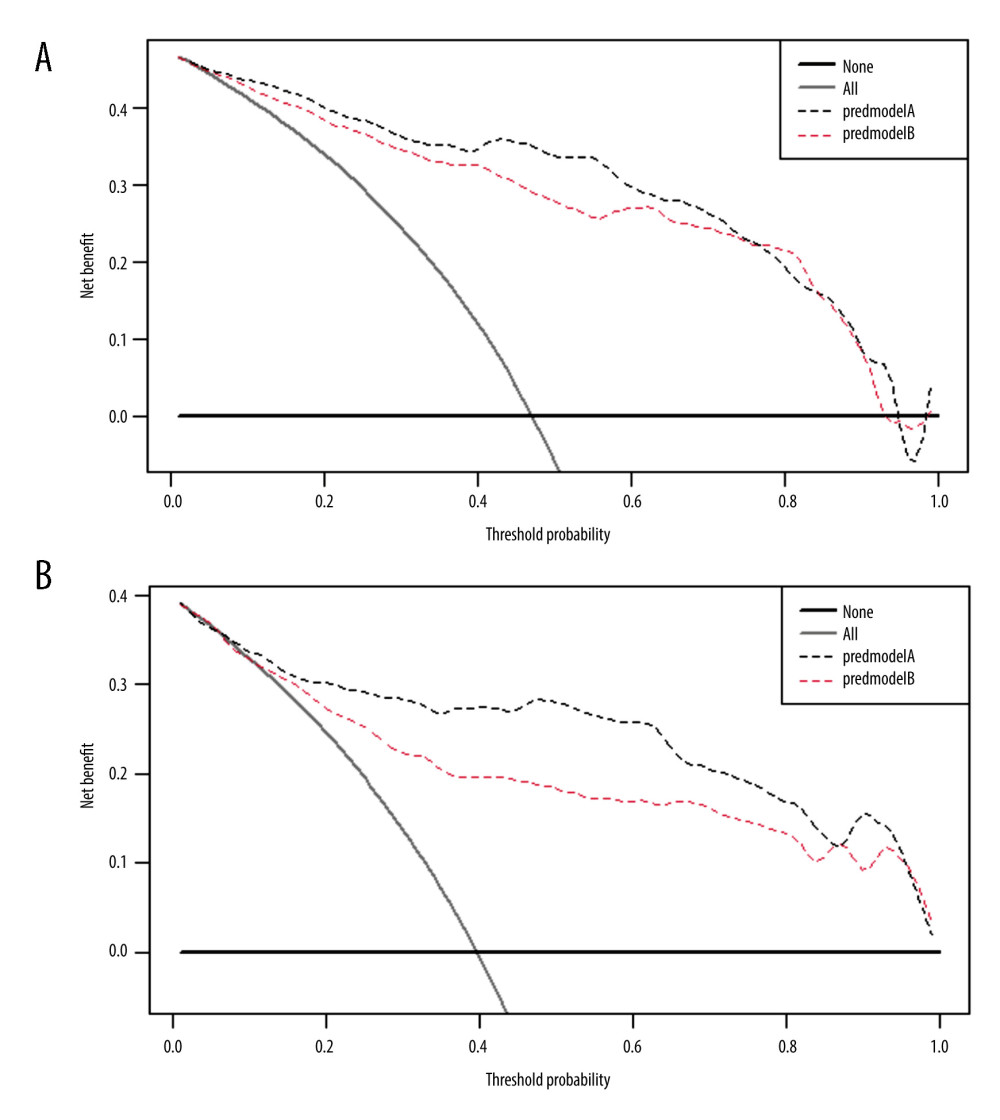
Tables
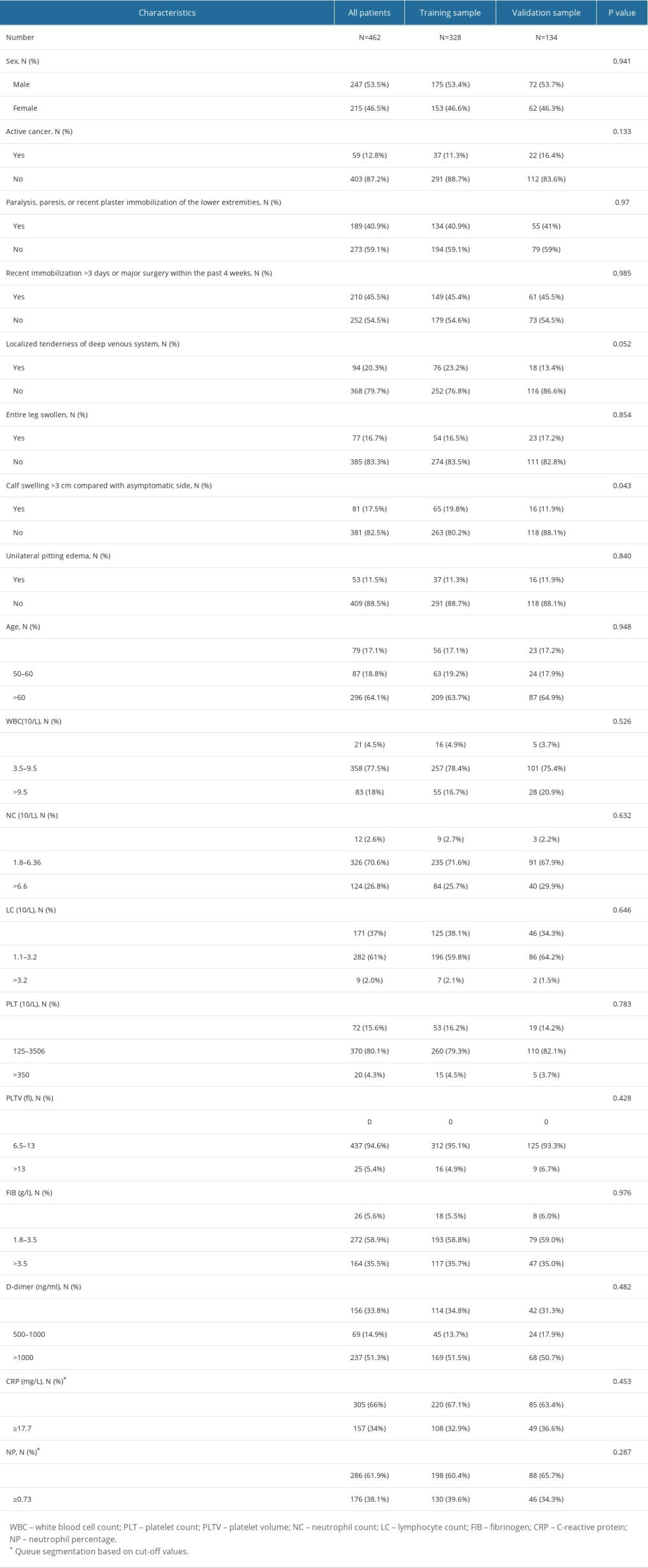
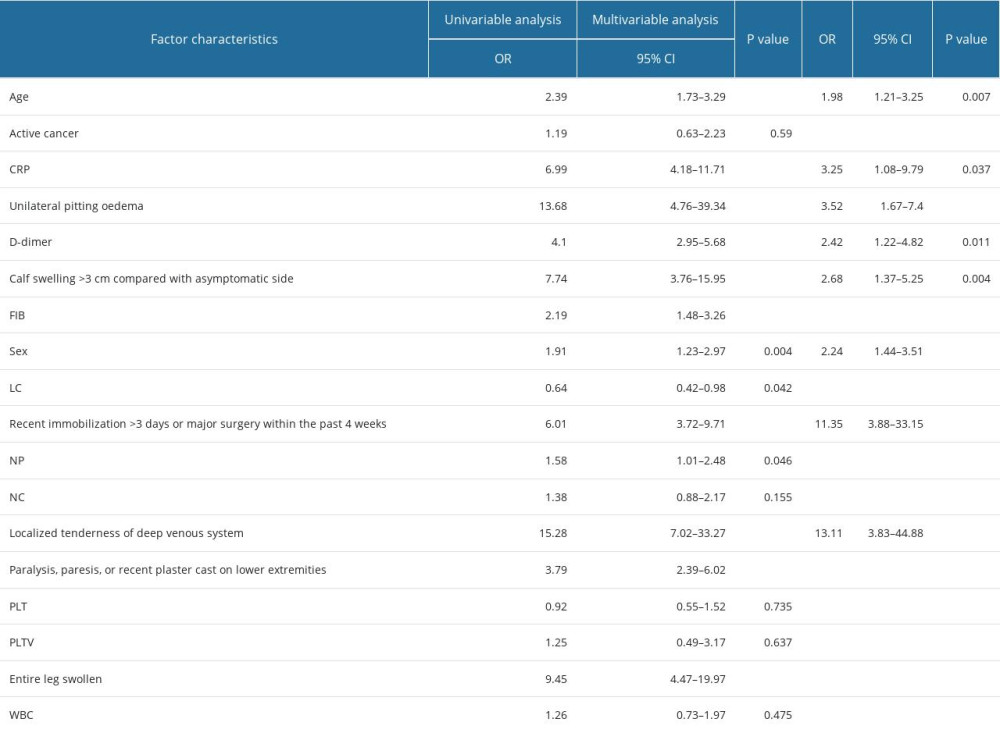
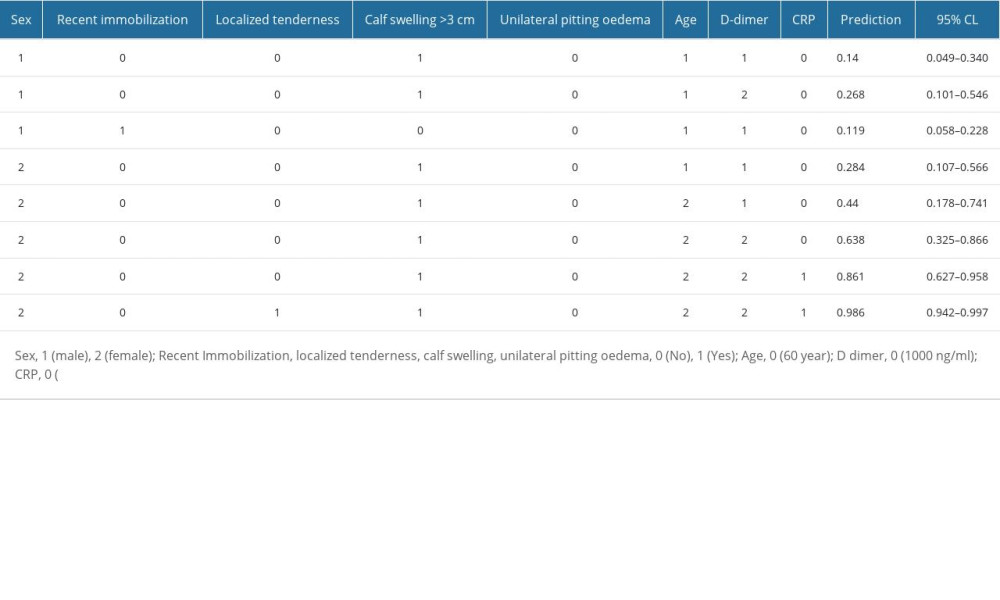
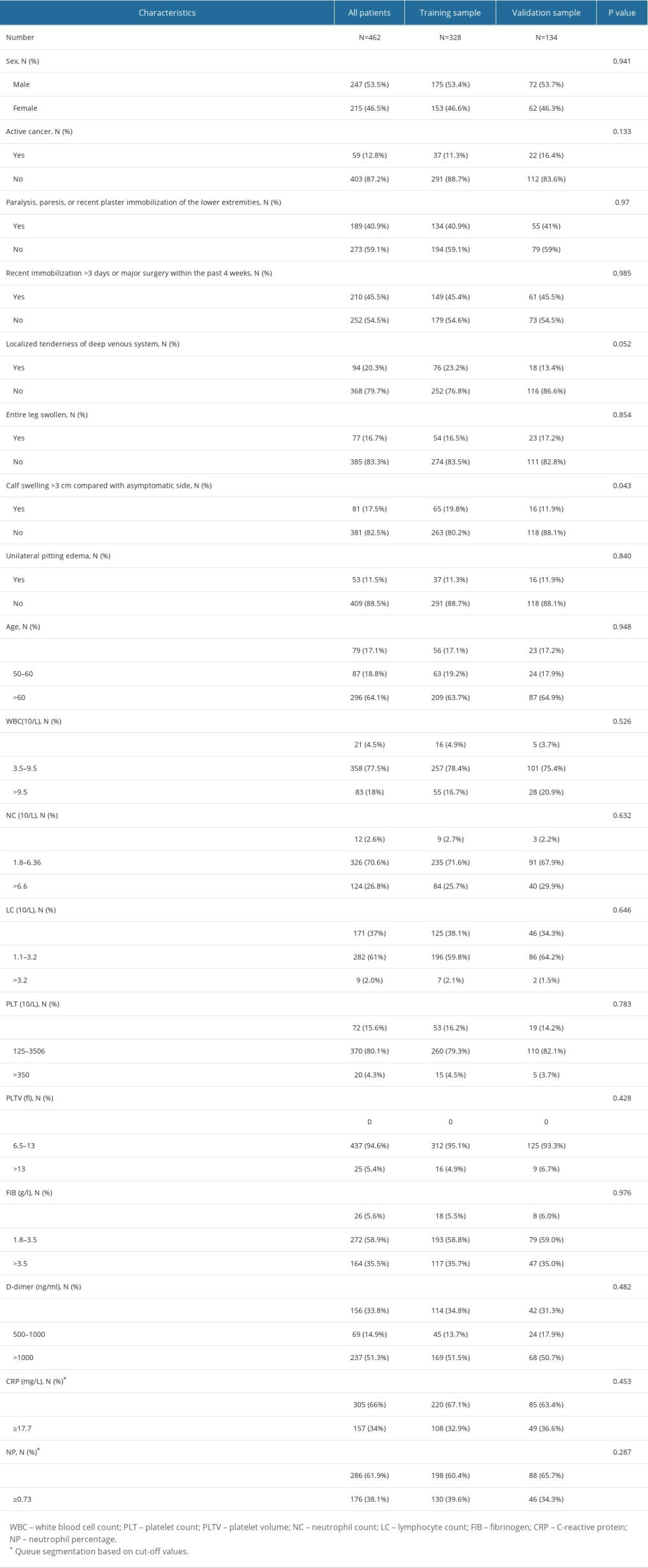
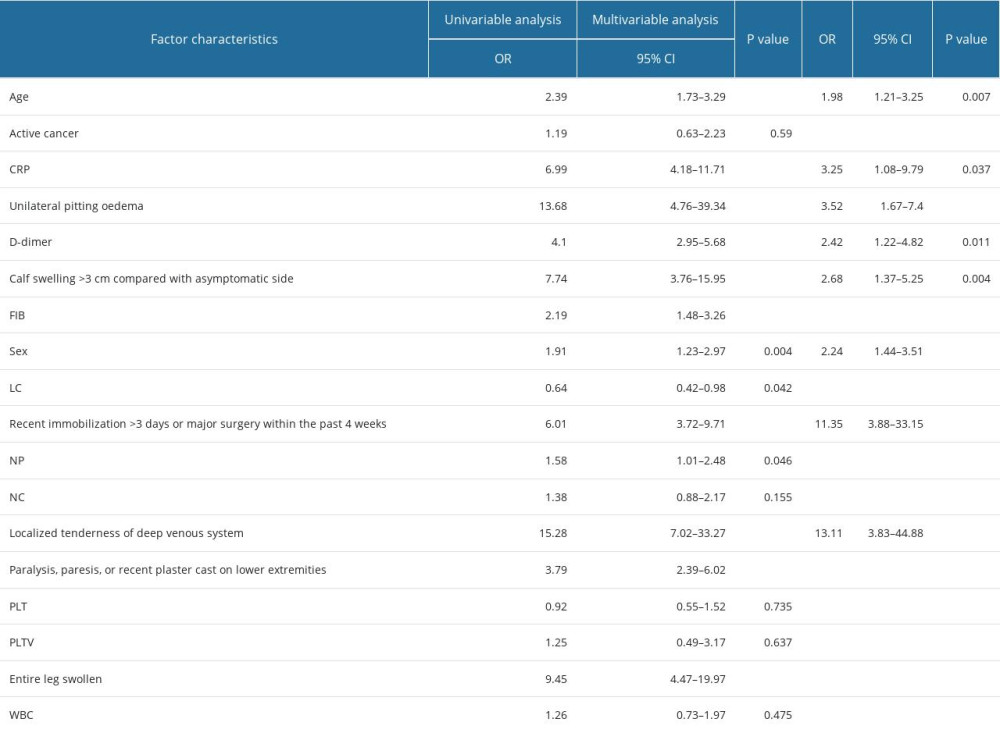
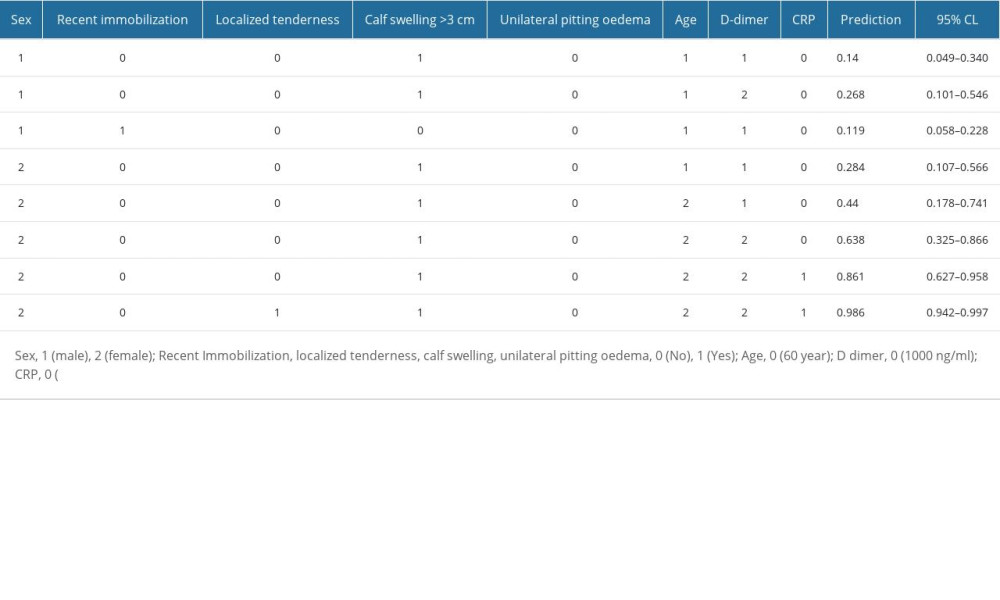
In Press
18 Apr 2024 : Clinical Research
Comparative Analysis of Open and Closed Sphincterotomy for the Treatment of Chronic Anal Fissure: Safety an...Med Sci Monit In Press; DOI: 10.12659/MSM.944127
08 Mar 2024 : Laboratory Research
Evaluation of Retentive Strength of 50 Endodontically-Treated Single-Rooted Mandibular Second Premolars Res...Med Sci Monit In Press; DOI: 10.12659/MSM.944110
11 Mar 2024 : Clinical Research
Comparison of Effects of Sugammadex and Neostigmine on Postoperative Neuromuscular Blockade Recovery in Pat...Med Sci Monit In Press; DOI: 10.12659/MSM.942773
12 Mar 2024 : Clinical Research
Comparing Neuromuscular Blockade Measurement Between Upper Arm (TOF Cuff®) and Eyelid (TOF Scan®) Using Miv...Med Sci Monit In Press; DOI: 10.12659/MSM.943630
Most Viewed Current Articles
17 Jan 2024 : Review article
Vaccination Guidelines for Pregnant Women: Addressing COVID-19 and the Omicron VariantDOI :10.12659/MSM.942799
Med Sci Monit 2024; 30:e942799
14 Dec 2022 : Clinical Research
Prevalence and Variability of Allergen-Specific Immunoglobulin E in Patients with Elevated Tryptase LevelsDOI :10.12659/MSM.937990
Med Sci Monit 2022; 28:e937990
16 May 2023 : Clinical Research
Electrophysiological Testing for an Auditory Processing Disorder and Reading Performance in 54 School Stude...DOI :10.12659/MSM.940387
Med Sci Monit 2023; 29:e940387
01 Jan 2022 : Editorial
Editorial: Current Status of Oral Antiviral Drug Treatments for SARS-CoV-2 Infection in Non-Hospitalized Pa...DOI :10.12659/MSM.935952
Med Sci Monit 2022; 28:e935952